Love and War
We have seen how even simple life forms, like bacteria, evolve to predict their environment, their internal states, and their actions. Both normativity (good versus bad) and basic feelings (like hunger, satiety, and pain) arise as consequences of prediction. However, the discussion so far has been framed in terms of a single-player game: how a “self” can evolve to enhance its dynamic stability in a world that is, if not hostile, at least indifferent. The food molecules a bacterium chases during chemotaxis are not running away; nor are chemorepellent molecules active aggressors. Chemotactic bacteria are modeling molecular concentration, but those small molecules they’re modeling (probably) aren’t modeling the bacteria in turn.
When it comes to wolves and rabbits, though, multiple agents are at work modeling each other—whether in skittish alarm (the rabbit) or with murderous intent (the wolf). Big-brained animals like us (and wolves, and rabbits) have detailed models of themselves, too, as well as of kith and kin—hopefully, with neither skittish alarm nor murderous intent. Such rich social, subjective, and “intersubjective” experiences seem far removed from the simple machinery of bff programs or bacteria. Yet I will argue that minds like ours are a natural consequence of the same evolutionary dynamics. In particular, minds arise when modeling other minds becomes important.
The need for such modeling goes a long way back. It relates to two major evolutionary transitions: the emergence of sexual reproduction, likely about two billion years ago, and of hunting, which became prevalent during the Cambrian explosion, 538.8 million years ago.
Because sexual reproduction is so ancient, first arising among our distant single-celled ancestors, its origins remain obscure. Sex may have been an integral part of the eukaryotic revolution, two billion years ago. 1 Since eukaryotes are the canonical instance of symbiogenesis, it seems fitting that sex is itself symbiotic: the female and male forms of a species depend on each other to reproduce, mutually benefitting through faster evolutionary learning. 2 (Hence, under most circumstances and all other factors being equal, a sexually reproducing species will outcompete one that reproduces asexually, even though each individual member of the asexual species faces fewer barriers to reproduction.)
For our purposes, though, sexual reproduction is relevant because it requires an organism to recognize one of its own, and, usually, to distinguish male from female and modulate its behavior accordingly. This is a big deal: when reproduction requires sex-selective cooperation or competition, others of the same species (and subtle differences between individuals) become an obligate part of one’s umwelt.
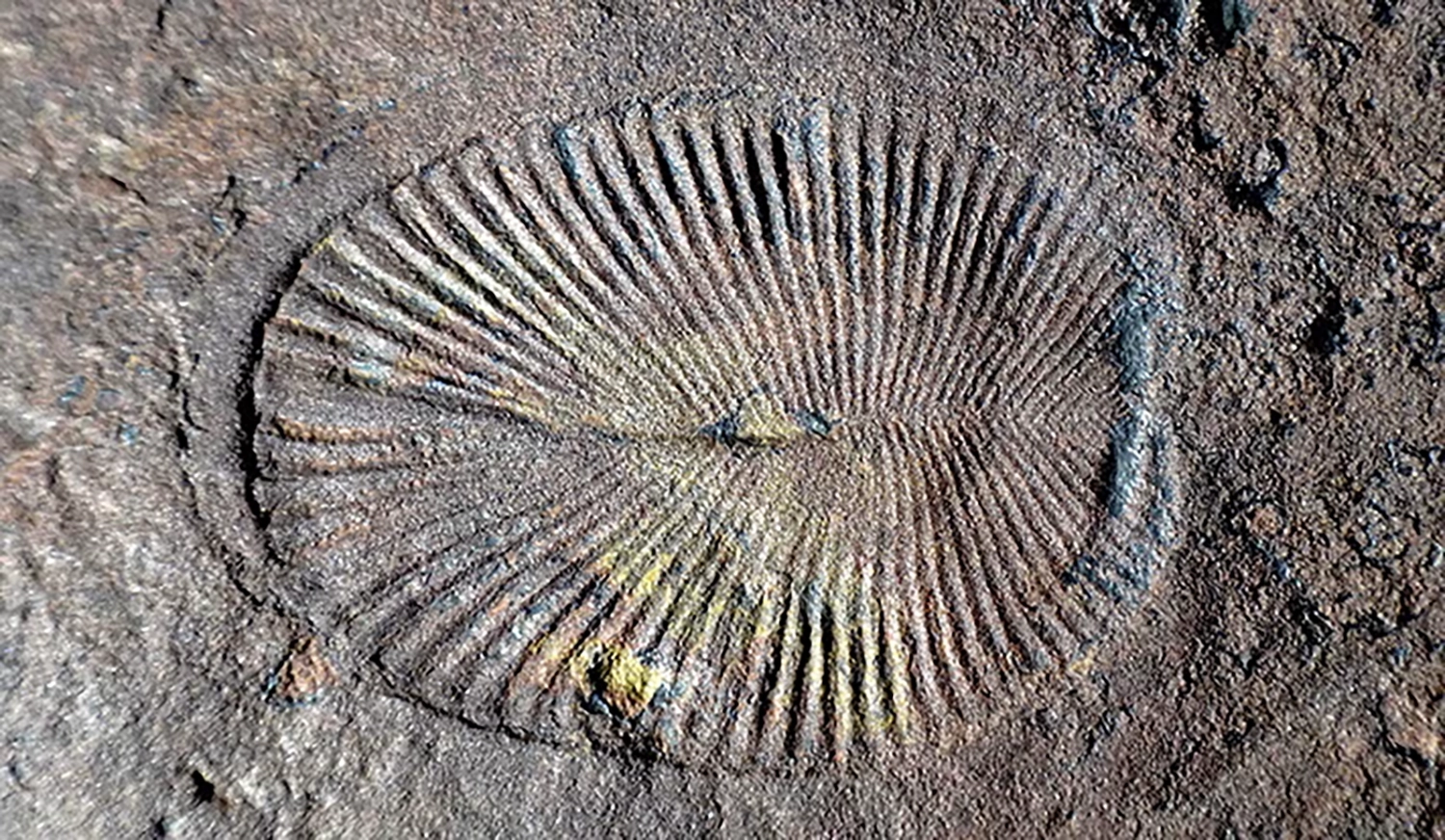
Fossil from the Ediacaran Biota
Now, let’s fast-forward a billion and a half years. While many aspects of the ancient fossil record remain controversial, there is broad consensus that pre-Cambrian life was dominated by soft-bodied organisms with a wide range of body plans and symmetries, from tubular to quilted to more amorphous. We can only guess how those ancient Ediacarans moved, but it seems likely that the behavioral boundary between plants and animals would have seemed indistinct, with many organisms either drifting on ocean currents or anchored to rocks, their fronds or appendages waving languidly in search of nutrients. Nervous systems during this period, when present at all, may have looked much like the distributed nerve nets of Hydra or comb jellies. 3
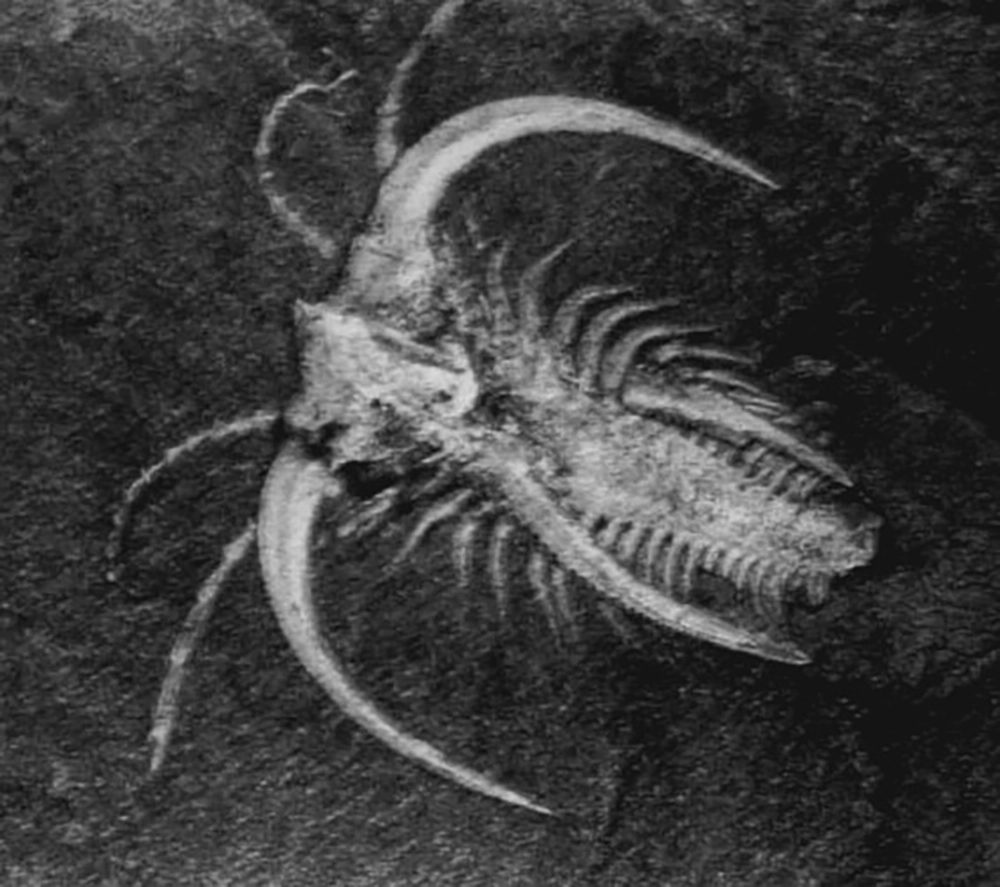
Marrella splendens fossil from the Burgess Shale, middle Cambrian
Then, the Cambrian explosion occurred: a sudden great diversity of marine creatures with protruding eyes, armored plates, fearsome claws, and sharp teeth. It’s impossible not to imagine them moving quickly and actively, hunting, escaping, killing and being killed. An arms race ensued, featuring a cascade of innovations and counter-innovations in the arts of attack and defense. For the first time, complex, centralized nervous systems would have been required to intelligently coordinate rapid, perceptually guided, purposive movement. 4 The (one-sided) joys of predation had been discovered.
In light of the earlier discussion of thermodynamics and its relationship to dynamic stability, it’s worth pausing to spell out the Darwinian motive for predation, even though it may seem intuitively obvious. Why do we eat, anyway?
Recall that life requires computation to reproduce (per chapter 1) and to respond to changes in its environment or internal state (per chapter 2). Computation, in turn, requires free energy—though recall also that this term is deceptive. Energy can neither be created nor destroyed; we don’t “use it up.” 5 Free energy really means a source of order, or low entropy.
Recall that in thermodynamics, the classic low-entropy system is a pressurized piston: a cylinder of air in which most of the gas molecules are concentrated on one side of a partition, leaving a partial vacuum on the other side. It would be extremely unlikely for this situation to arise spontaneously—like randomly bouncing billiard balls suddenly forming a triangle at one end of the table—but with the piston locked in place to prevent the gas from redistributing itself more randomly, the pressure differential can be maintained.
This low-entropy configuration is like a loaded spring, or a cocked gun. The moment that piston is allowed to move, it will do so with force, until the pressures on both sides equalize—that is, until entropy is again maximized. That would “use up” the free energy, even though the total amount of actual energy in the system (proportional to the summed squared velocities of all of the gas molecules) has remained constant. When Newcomen and Watt harnessed the piston’s movement to work a pump, or spin a loaded crankshaft, the molecules would slow down accordingly, cooling the gas.
Now, remember that once life takes hold, it creates predictability and order; its entropy plunges. That, too, requires work. It also means that an organism itself is a kind of pressurized piston—or loaded spring, or cocked gun. A wonderful source, in other words, of free energy. (The fatty bits, especially; all those delicious carbon-hydrogen bonds!) Thus, predation: a life form using the order, or “negative entropy,” stored in the body of another life form to do the work of maintaining or increasing its own order or negative entropy. 6
There are conflicting interests here. In the best case (for the victim), the predator will just take a harmless little nip or sip—that’s how fleas, ticks, flies, and mosquitoes work. We still resent them; grooming behaviors among primates, and the swishy tails on horses, evolved to discourage such micro-predation. (And partly in response, flies have become lightning-quick, mosquitoes have evolved to inject anesthetics, and ticks have become tenacious flesh-burrowers.) As for predation of the kind that comes in larger, life-ending bites—well, avoiding it at any cost immediately rises to the top of any prey animal’s to-do list.
At first blush, the two great revolutions I’ve just described—sex and predation—seem to have as little to do with each other as love and war. However both love and war require that you recognize and form internal models of other living things in the pursuit of dynamic stability. They require, in other words, effective prediction of something that is itself a predictor. 7
We’ll get to love later in the book. Here, though, we’ll focus on warfare—the original “killer app”—from a computational perspective. Let’s return briefly to nineteenth century London.
Killer App
Babbage struggled to drum up financial backing for his Analytical Engine. The market for Jacquard-woven textiles was obvious, but who needed industrially mass-produced “analytical formulæ”? To sell the idea of mathematical tables as a popular commodity, he resorted to folksy examples, such as “the amount of any number of pounds from 1 to 100 lbs. of butchers’ meat at various prices per lb.” 8
Price tables for the village butcher? Hardly. A moment’s reflection will make it clear that small tradesmen wouldn’t have been a viable customer base for a hulking industrial machine like the Analytical Engine.
State administration, which Prony had bet on, was closer to the mark; the information needs of bureaucratic governments were on the rise. 9 Still, it was too early. The French government defunded Prony’s project long before it was complete.
The real killer app was warfare. The British Army and Navy would have been Babbage’s obvious backers, and ultimately it was their lack of investment that doomed his enterprise.
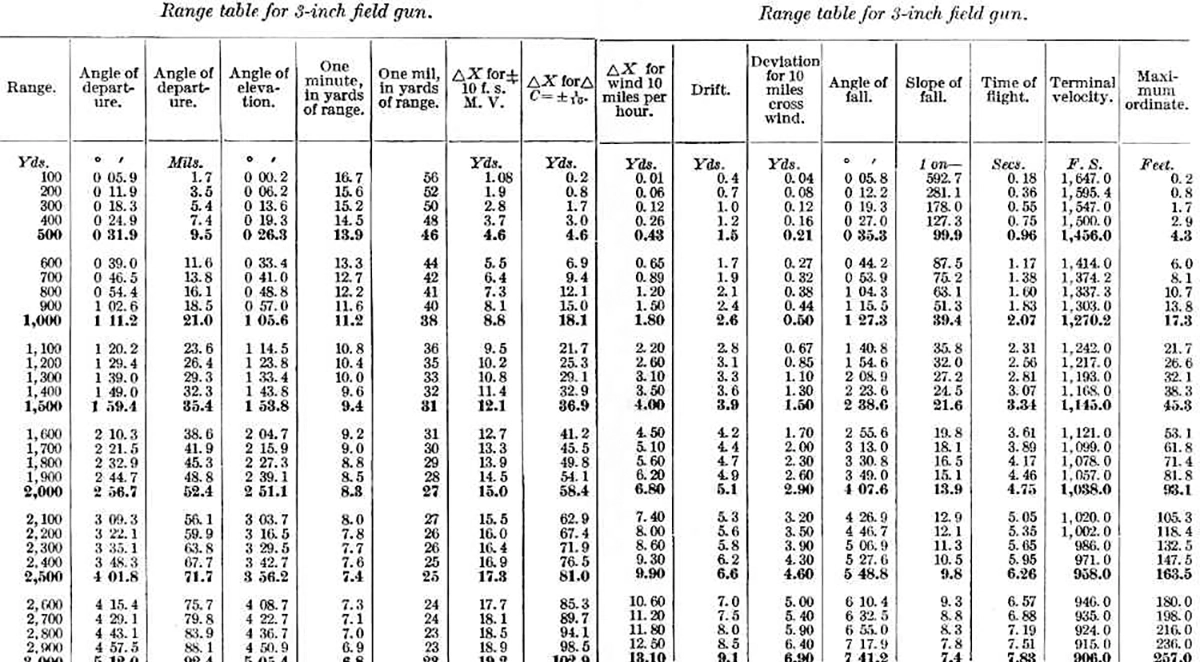
Range tables for US 3-inch field gun, models 1902–1905, 15-lb. projectile
The artillery table was already a paradigmatic product of human computation by the turn of the nineteenth century. A new table was needed for every big gun, including corrections for factors like altitude, wind, and barometric pressure. With every major world conflict from the Napoleonic Wars (1803–1815) onward, gunnery became increasingly important, and, with it, tabulation. And doing the calculations by hand took a long time.
World War I artillery
By World War I, the first fully industrialized large-scale conflict, both the Allies and the Central Powers were making extensive use of complex tables. Artillery fire was often planned days in advance, and its accuracy became crucial for supporting infantry advances. Computation had become a bottleneck in warfare, and, more than any other single factor, this was what finally motivated serious investment in automatic computing between the World Wars.
As weapons production for World War II began ramping up, the University of Pennsylvania’s Moore School of Electrical Engineering hired at least two hundred women to work on artillery tables. Their methods would have been largely familiar to Babbage or Prony. But, spurred by the war effort, technology was advancing at breakneck speed. Six of the Moore School’s women were selected to become the programmers of the ENIAC. 10 This first fully general, programmable computer had been designed to automate artillery tabulation. 11
Short film about the ENIAC, 1946
By the time the ENIAC became operational, in December 1945, priorities had changed. The Germans and the Japanese had been defeated, but Cold War brinkmanship picked up right where World War II had left off. The new computer’s first substantial program was a simulation of the “Super Problem,” exploring the feasibility of a hydrogen bomb. 12 The math required was a lot harder than calculating ballistic trajectories, and the machine’s thousands of vacuum tubes burned out frequently, requiring near-daily repair. This incentivized rapid improvements in the hardware, kickstarting what would later be known as Moore’s Law. 13
The early computers were a long way from anything we have today. It’s sobering to consider how many years of capital-intensive incubation within the military-industrial complex were needed before the technology had become sufficiently cheap, reliable, and miniaturized to rouse real interest from the private sector, let alone the village butcher.
IBM’s 701 mainframe, announced to the public on May 21, 1952 and originally dubbed the “Defense Calculator,” became the first computer commercially available in the US. There’s an apocryphal quote, usually attributed to Thomas J. Watson Jr., IBM’s president, from a stockholders meeting in 1953, to the effect that he believed there was a worldwide market for only five computers. This isn’t quite true; Watson really said that, when IBM had drawn up the plans for the 701 and toured them across the country to “some twenty concerns” that they thought “could use such a machine,” they had assumed they’d only get five orders and were astonished to get eighteen. 14
At first, general-purpose computers were not designed to work in real time. Like the human computers they replaced, they supported the war effort using batch processing. Such computing was a stately, offline affair. You submitted your job, and went to get a coffee … or, more likely, take a nap while technicians nursed the job along, replacing tubes, clearing punch-card-reader jams, and swapping out spools of magnetic tape. Running on the ENIAC, bff would have taken centuries to achieve full-tape replication. By 1952, on the IBM 701, it would still have taken years, running nonstop, at a cost of millions of (today’s) dollars. 15
Promotional clip of the IBM 701, 1953
The ’50s did see some early experiments in interactive architectures. Project Whirlwind, operational in 1951, was initially designed for flight simulation, and later became the heart of the US Air Force’s SAGE air-defense system. 16 Real-time computing had become important due to the development of radar and related radio-signaling technologies, effectively allowing one machine to physically detect another machine—at least one of which might be moving through space at high speed and with lethal intent.
Identify Friend or Foe (IFF) systems soon followed, using encrypted signaling to allow radar dots to be annotated when a bogey was “one of ours.” This in turn created an incentive to hack an enemy’s IFF system, spoofing the “friend” signal to sneak into enemy territory. A game of technical one-upmanship ensued, not unlike that of the Cambrian explosion.
Still more Cambrian was the rapidly increasing speed, precision, and deadliness of the military hardware. Jet fighters, missiles, and anti-missile defenses proliferated. GPS was invented in large part to make autonomous weapons guidance possible, as it was obvious that high-speed warfare would soon render keeping a human “in the loop” impossible; the precision and response time needed were superhuman. Even the G-forces incurred by aerial maneuvers soon began to exceed human endurance. 17 Robots would need to close the sensorimotor loops of these new weapons systems.
Behavior, Purpose, and Teleology
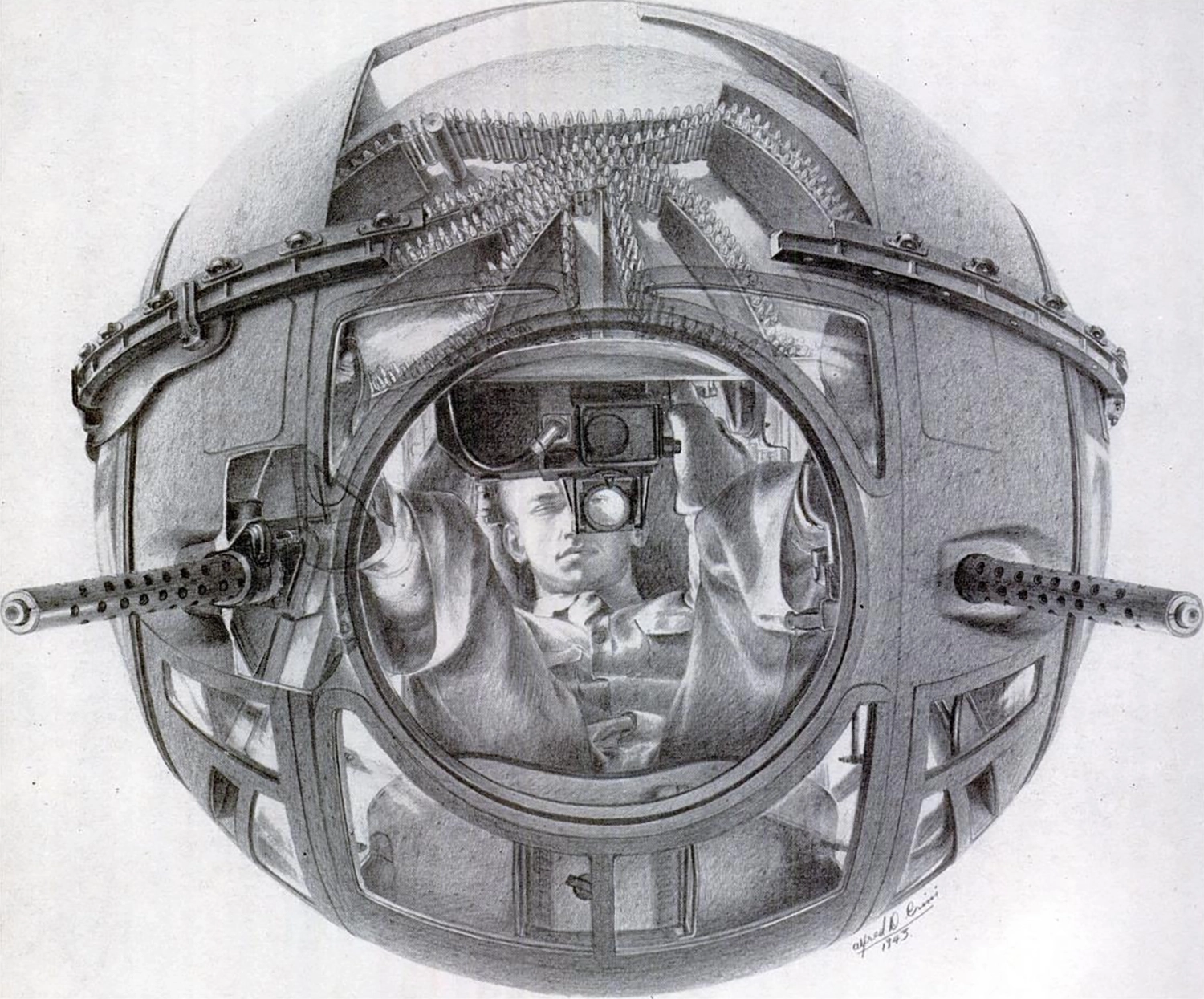
World War II–era Sperry ball turret drawn by Alfred D. Crimi, 1943
Thus, the field of cybernetics was born, so named in 1947 by MIT mathematician Norbert Wiener, physiologist Arturo Rosenblueth, and their colleagues. 18 Unlike batch computing, cybernetics was all about real-time feedback loops involving continuous time-varying signals like position, velocity, and thrust. Early proto-cybernetic technologies included electromechanical gun turrets and automatic bomb-sighting engines used to correctly time the dropping of munitions from a plane to hit a target, often using analog cams and gears. 19
The cyberneticists took on the challenge of generalizing such systems to aerial dogfighting, in which the target is not stationary. 20 In the presence of noise and uncertainty, such problems can be formulated using information theory, a field where Wiener made important early contributions. Under the assumption that the “brain” of a targeting system is linear, meaning that its outputs are weighted sums of its inputs, he also derived elegant theoretical results in optimal filtering and control. (Bacterial chemotaxis, described in chapter 2, can be modeled using the same math. 21 ) These optimal linear theories are both useful for building weapons systems and, under many real-life conditions, can be hard to improve on: when the goal is clear and unchanging, everything is happening very fast, and measurements are noisy, there isn’t time for nonlinearity to matter so much.
In high-speed, goal-directed, and similarly adversarial natural contexts, such as a bat or dragonfly closing in on prey, animal brains can also carry out something close to cybernetically optimal linear modeling. 22 The same holds when you reach for an object or catch a ball. 23
Bat using sonar to hunt moths
Balls don’t actively try to escape you—at least outside the Harry Potter universe. A moth, however, behaves like a real-life “golden snitch” when a bat is closing in for dinner. 24 It can’t fly as fast as a bat; its brain and sensory apparatus are outmatched too. But it can try evasive action. The evasions must be genuinely unpredictable, because randomness is the moth’s only cybernetic advantage. The bat, with its much larger brain, would preempt any more coherent plan the moth could dream up. So, while the bat applies something close to optimal prediction tailored to the moth’s flight statistics, the moth takes advantage of its low mass to flutter around chaotically, rendering those statistics as blurry as possible. Instead of a smooth, predictable curve, the resulting flight pattern is a tortuous “random walk” occupying a volume in space known affectionately as the “Wiener sausage.” (For real. 25 )
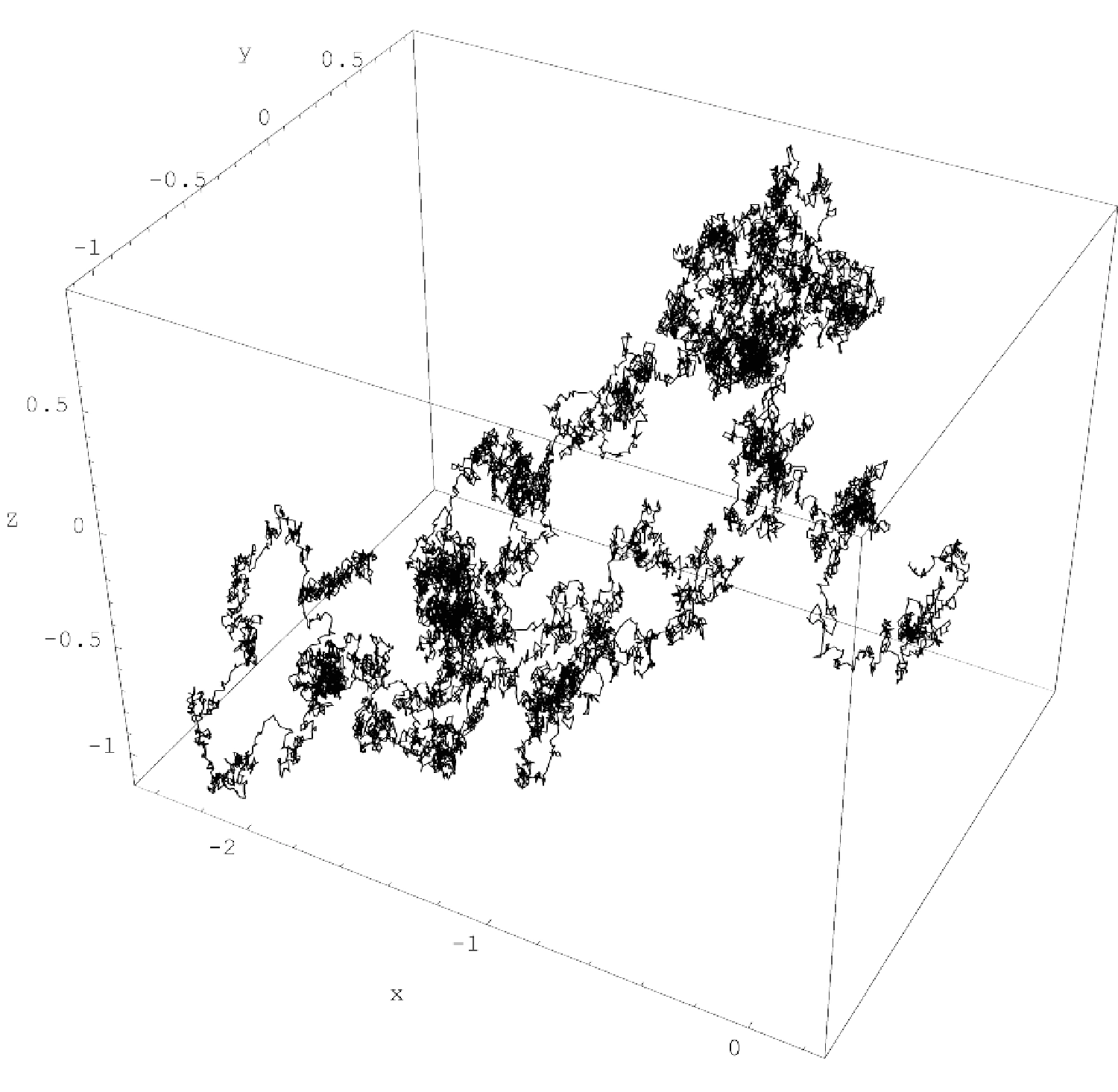
Simulation of a particle diffusing randomly in 3D, its path approximately encased in a “Wiener sausage”
As you will know if you’ve ever tried—and failed—to catch a seemingly not-that-fast moth, these Wiener-sausage tactics work, at least up to a point. The moth implements them with a biological random-number generator, likely based on a combination of mechanical instability in the wings and random neuronal activity. If you’re wondering how neuronal activity can be random, keep in mind that it always is, a little bit. The biochemical events leading to neural firing include ion channels opening and closing in the cell membrane, and the release and capture of synaptic vesicles containing neurotransmitters. The timing of these events is never quite precise, because they’re contingent on the same random molecular interactions that drive all cellular activity. If a neural circuit is wired to amplify that noise, then, like the random-number instruction of Turing’s Ferranti Mark I computer, the result will be a computationally usable random signal. 26
Ample evidence suggests that prey species like moths use such circuits to generate chaotic behavior; startled cockroaches, similarly, use them to scurry at random. 27 If randomness is the low road to cybernetic unpredictability, though, there is also a high road: becoming smarter than the entity trying to predict you, and predicting that entity’s predictions. We’ll explore this brainier strategy in chapter 5.
But first, let’s take the full measure of cybernetics—what it got right, what it got wrong, its untimely demise, and its enduring influence. As formulated by Wiener, cybernetics was the field we now call Artificial Intelligence, and more—it was the hallmark of all complex systems, a grand unifying theory of the biological, the technological, and even the sociological. 28
The simplest cybernetic system is something like a humble thermostat, a feedback mechanism that turns the heat (or air conditioning) on and off to maintain a constant temperature. Warm-blooded animals, of course, do just that, and living systems in general must use feedback to “homeostat” (that is, to regulate) their internal state. Construed broadly, homeostasis is what being alive is all about. The point of eating, for example, is to maintain the body’s store of free energy, and similar purposive arguments can be made for all of our primary drives. Our secondary drives, such as the desire for praise or prestige, can in turn be formulated as “instrumental” to those primary drives. Homeostasis is thus our old friend, dynamic stability, implemented purposively.
Complex animal behaviors may seem a long way from those of a thermostat, but consider that a truly optimal thermostat will not only switch on or off in response to fluctuations in the temperature now, but also on the basis of its prediction of the future. If, for instance, the sun is about to rise and start rapidly warming your house, this ought to affect your home thermostat’s strategy.
Generalizing this observation in a 1943 essay entitled “Behavior, Purpose, and Teleology,” 29 Wiener, Rosenblueth, and computer pioneer Julian Bigelow perfectly articulated the “predictive brain” hypothesis in cybernetic terms (though they did not coin the word for several more years):
All purposeful [or “teleological”] behavior may be considered to require negative feed-back [which] may be extrapolative (predictive) […]. A cat starting to pursue a running mouse does not run directly toward the region where the mouse is at any given time, but moves toward an extrapolated future position. […] The cat chasing the mouse is an instance of first-order prediction […]. Throwing a stone at a moving target requires a second-order prediction […]. It is probable that limitations of […] the central nervous system […] determine the complexity of predictive behavior […]. Indeed, it is possible that […] the discontinuity [between] humans [and] other high mammals may lie in that the other mammals are limited to predictive behavior of a low order, whereas man may be capable potentially of quite high orders of prediction.
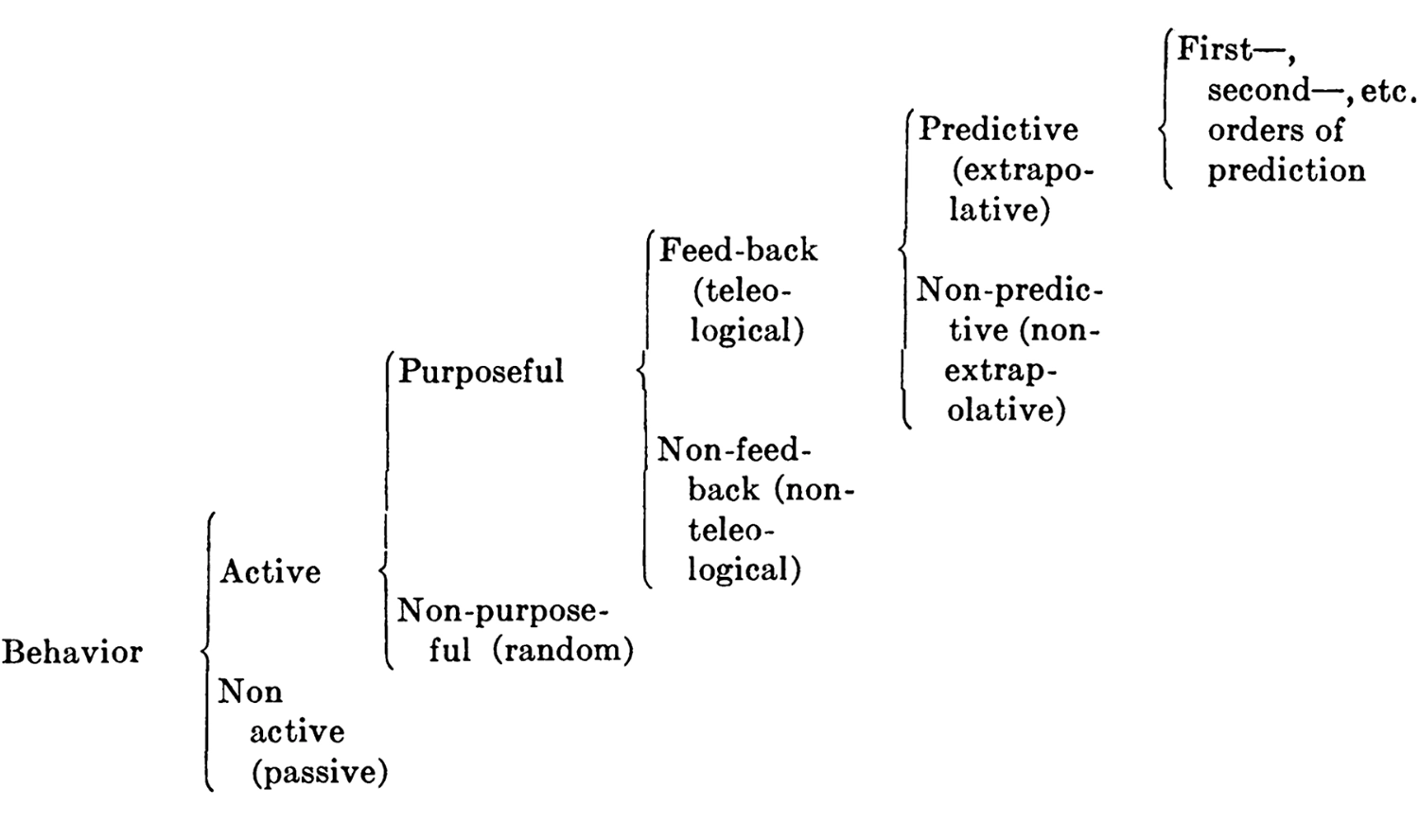
Classification of behaviors from Rosenblueth, Wiener, and Bigelow 1943
The authors also pointed out that, from this behavior-focused perspective (that is, without worrying about how the predictive computation is implemented), purposive machines and living systems are alike, “regardless of the complexity of [their] behavior,” adding that “examples […] are readily found of manmade machines with behavior that transcends human behavior.”
This was true of machines even in 1943, before general-purpose computing; it was even true in 1843, or there would have been no Industrial Revolution, though few machines back then were teleological or purposive in the cybernetic sense. A notable exception is the centrifugal governor, first invented by Christiaan Huygens in the seventeenth century, then adapted by James Watt in 1788. Watt’s version controlled the amount of steam going into a steam engine using a valve coupled to whirling weights driven by the engine, creating a negative (or homeostatic) feedback loop to regulate the engine’s speed.
In invoking teleology, Wiener and colleagues were playing with fire, both in the philosophical and scientific communities. Materialism and reductionism had given teleology a bad name, relegating it to a quasi-religious belief. After all, if, given initial conditions, the (entirely knowable) dynamical laws of physics fully determine what will take place at the next moment in time, what role could teleology or purposive action possibly play? 30
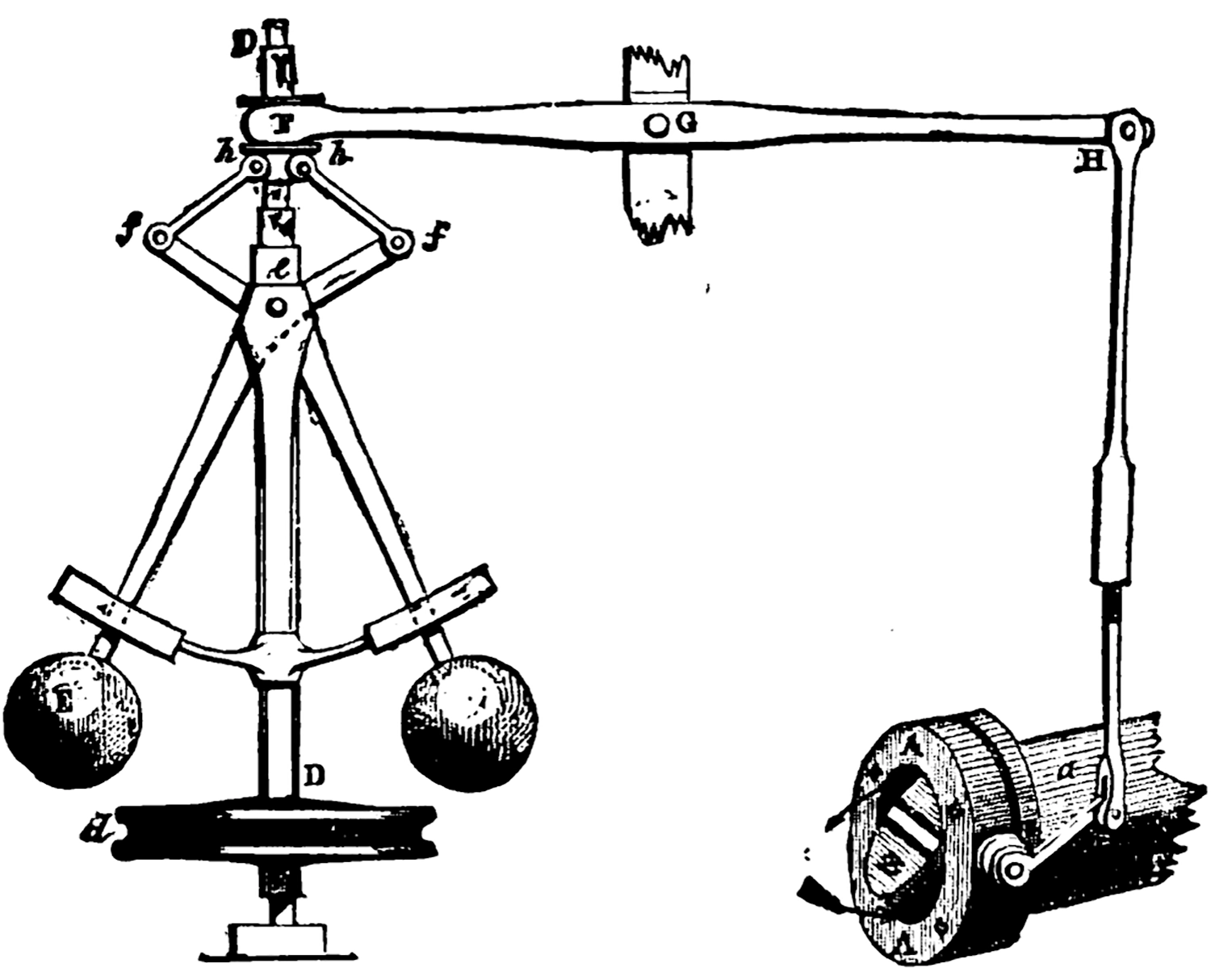
A centrifugal governor: the balls swing out as speed increases, closing the throttle
Beyond stipulating goals and purposes, cybernetics posited agents that predict the future and bring that future about—the same apparent reversal of causality we encountered in chapter 2. The cyberneticists’ insight, both trivial and profound, was that predictive negative-feedback loops are sufficient to give an apparatus (or organism) purposiveness … without violating the laws of physics. The Huygens-Watt centrifugal governor, for instance, is a simple machine whose workings are fully described by Newtonian physics. Yet it also has a goal: it manipulates a steam engine’s intake valve to regulate the engine’s speed. In doing so, it “creates” causes in order to bring about future effects.
The apparent paradox of backward causality resembles the apparent paradox of an apparatus (or organism) building a copy of itself. In that case, von Neumann realized that the solution lay in the apparatus having an internal model of its own structure, and using that model to guide construction. Wiener and colleagues realized that the solution to their problem lay in the apparatus having an internal model of (part of) the world, and using that model to guide behavior.
These are flip sides of the same coin. In both cases, the models are computational; and in both cases, their purpose is to continue to exist through time, whether by growing, by preserving the integrity of the self, or by replicating.
Von Neumann and Wiener published their insights within a year of each other, though, at the time, the intellectual kinship between them may not have been fully appreciated.
Negative Feedback
Cybernetics got many things right, especially as compared with the symbolic, programming-based approach to AI championed by Wiener’s detractors. For starters:
- It embraced continuous values and random processes.
- It was based on learning (that is, modeling) probability distributions, rather than executing hand-engineered code reflecting a programmer’s intuitions.
- It advanced the idea that agency (or purposiveness, or teleology) is fundamental to intelligence, and to life more broadly. 31
- It was consistent with the neuroscientific consensus that emerged soon after McCulloch and Pitts’s 1943 paper, when it was understood that neurons do not implement propositional logic using Boolean values.
- It focused on the continuity between intelligence and living systems more generally, rather than imagining that intelligence is a purely logical construct in the Leibnizian tradition—and therefore the exclusive province of symbolically minded humans. As a corollary, cybernetics presumes that bats, dragonflies, and even bacteria are also intelligent.
- It emphasized the importance of behavior over mechanism, noting that the same models could be computed in radically different ways—for instance, in standard Turing Machine–like computers, “largely by temporal multiplication of effects” given “frequencies of one million per second or more,” or in biological systems, by “spatial multiplication,” that is, massive and non-deterministic parallelism. 32
This last point may sound reminiscent of B. F. Skinner and radical behaviorism, a school of thought that has been pilloried as brutally reductionist, or as denying the existence of mental states. 33 A fair assessment of behaviorism would take us too far afield, but suffice it to say: Wiener and colleagues were making a point about the fundamentally computational nature of the brain or mind. Hence, its multiple realizability, or platform independence, is underwritten by the universality of computation, per Church and Turing. This is the same kind of “behaviorism” that led Turing to formulate the Imitation Game in terms of behavior, rather than insisting on any specific brain mechanism.
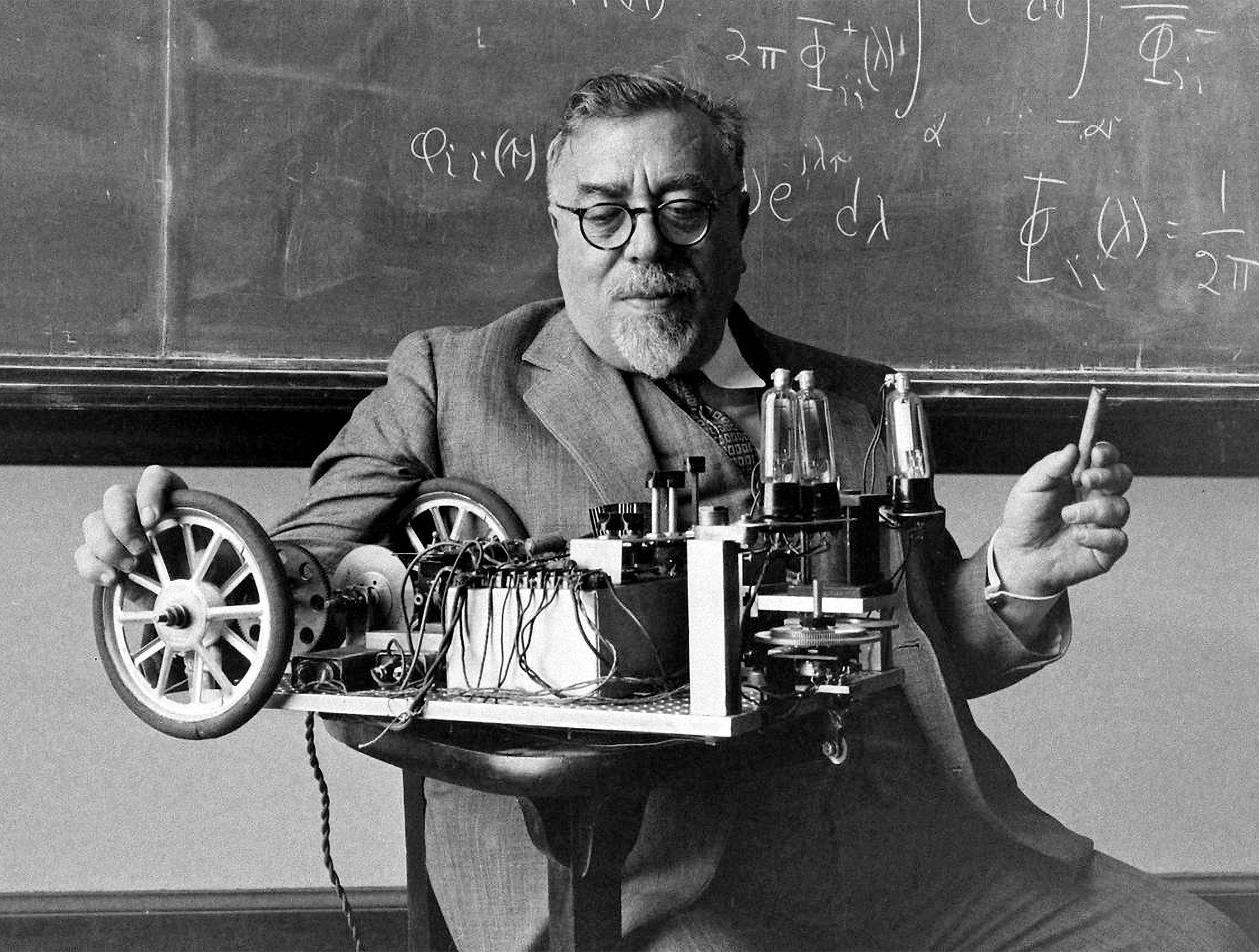
Norbert Wiener at MIT with Palomilla, 1949
Cybernetics also got some things wrong. Its greatest flaw was in overpromising: there was a vast gulf between Norbert Wiener’s self-promoting grandiosity and the engineering realities of his day. The theory was so general that it was nearly tautological, yet practical demonstrations using mid-twentieth-century technology were underwhelming. Neither was Wiener much of an engineer; he was more at home at a blackboard than in a machine shop. An idea for a cybernetic antiaircraft-gun controller, which he had insisted would prove crucial to the war effort, remained vaporware.
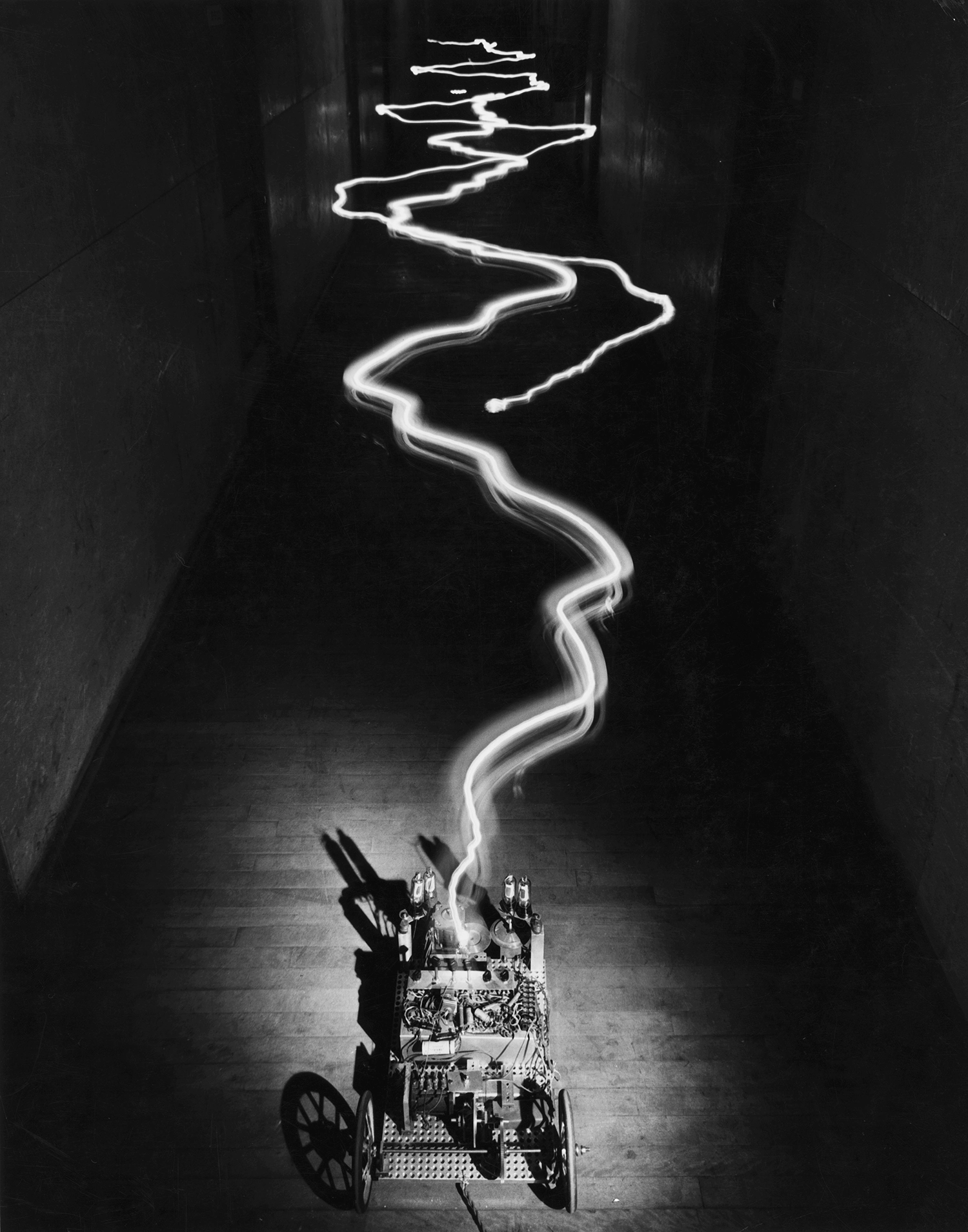
Time-lapse exposure of Norbert Wiener’s Palomilla following a flashlight down a hall.
In 1949, Wiener’s students at MIT cobbled together a motorized cart with wheels driven by feedback loops involving two photocells. Wired up one way, it was christened Palomilla, Spanish for “moth,” and could trundle bumpily along a corridor, more or less following a flashlight beam. Switching the wires turned it into a light-averse “bedbug.” Cranking up the feedback gain made it oscillate with something like Parkinsonism or intention tremor. But beyond the tight feedback loops of missile control systems (which did what Palomilla did, marginally more reliably 34 ), real-life applications were elusive. Why were these demos so lame?
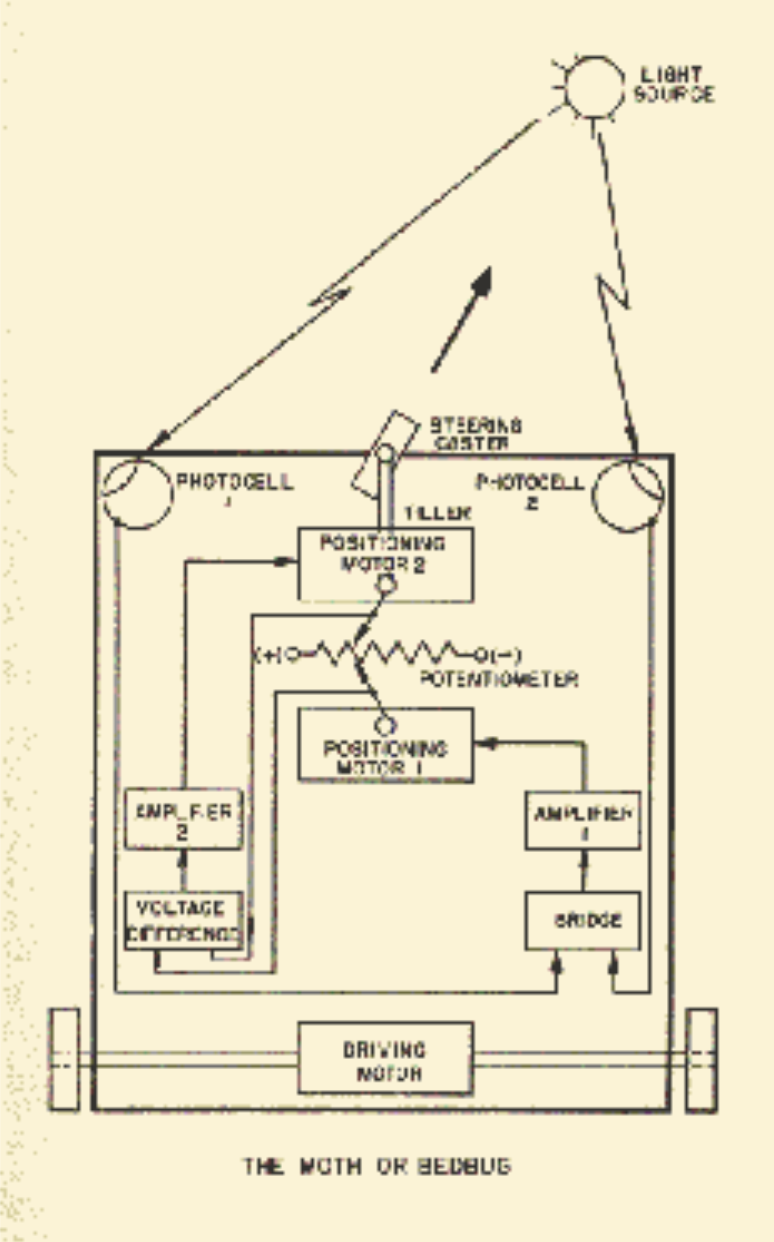
Schematic diagram of Palomilla
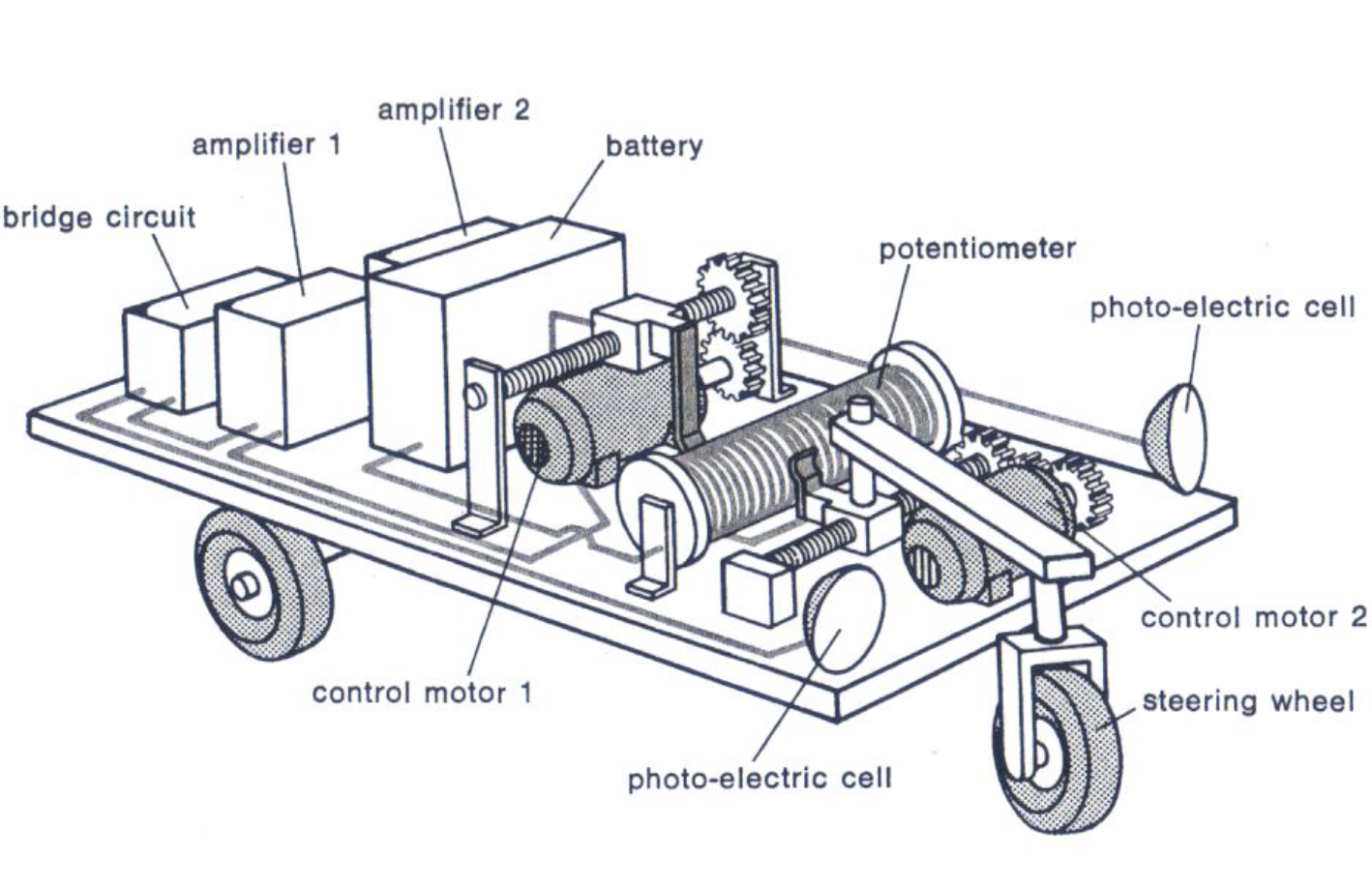
Isometric view of Palomilla
Wiener could easily write down a feedback-response function that would stand in for the totality of an organism’s behavior. He could then proceed to expand that function formally into an infinite series of higher-order terms. He could even derive closed-form solutions for the linear parts, given a simple goal like playing an optimal game of chase with an opponent whose movements could be analyzed statistically. Yet the higher-order terms remained out of reach. Exploding numbers of parameters and the difficulty of characterizing higher-order goals made it unclear how further progress could be made—especially given the feeble computational power available.
Access to massive computation would not have immediately solved the problem, either, because major conceptual gaps remained. Cyberneticists had largely swept memory and learning under the rug, along with anything resembling individual behavior. Lofty rhetoric aside, what cybernetics offered in practice was a cartoon of the purely instinctual behavior of a simple organism performing a single low-dimensional sensorimotor task in an unchanging niche. A thermostat, basically.
Real moths and bedbugs have a vastly richer behavioral repertoire than the Palomilla. Even chemotactic bacteria do. Those pesky feedback functions were black boxes where seemingly infinite complexity hid. In theory, more or less any function could be approximated with a series expansion, but, in practice, it was hard to see how anything resembling thought, perception, or action—let alone anything like psychology or a “self”—could arise merely by adding more terms to the series.
Good Old-Fashioned AI or GOFAI, based on hand-written programs instead of cybernetics, may have been cheating, but at least its canned responses could be substantive. In one famous example, MIT professor Joseph Weizenbaum created a chatbot called ELIZA, which, given a modest collection of grammatical rules, could simulate a therapy session.
Joseph Weizenbaum’s ELIZA
The year was 1964—the heyday of psychologist Carl Rogers’s “person-centered therapy.” Rogers believed in the power of patients talking themselves through their problems, and avoided ever passing judgment; he called this “unconditional positive regard.” Hence, his method consisted mainly of listening empathically and encouraging the patient to carry on. ELIZA could approximate this kind of engagement by using heuristics to guess at key words or phrases in the dialogue and ask followup questions about them, or, if stumped, to fall back on restatement or asking a more general question.
As Weizenbaum put it, “This mode of conversation was chosen because the psychiatric interview is one of the few examples of […] communication in which one of the participating pair is free to assume the pose of knowing almost nothing of the real world. If, for example, one were to tell a psychiatrist ‘I went for a long boat ride’ and he responded ‘Tell me about boats,’ one would not assume that he knew nothing about boats, but that he had some purpose in so directing the […] conversation.” 35
In a 1966 paper, Weizenbaum reproduced an ELIZA dialogue he described as “typical”; the machine’s responses (in caps) are accurate, though the exchange is cherrypicked to avoid bloopers:
Men are all alike.
IN WHAT WAY?
They’re always bugging us about something or other.
CAN YOU THINK OF A SPECIFIC EXAMPLE?
Well, my boyfriend made me come here.
YOUR BOYFRIEND MADE YOU COME HERE
He says I’m depressed much of the time.
I AM SORRY TO HEAR YOU ARE DEPRESSED
It’s true. I am unhappy.
DO YOU THINK COMING HERE WILL HELP YOU NOT TO BE UNHAPPY
I need some help, that much seems certain.
WHAT WOULD IT MEAN TO YOU IF YOU GOT SOME HELP
Perhaps I could learn to get along with my mother.
TELL ME MORE ABOUT YOUR FAMILY
… and so on. 36
It would be hard to argue that a few hundred stock responses with their blanks filled in by echoing back user-supplied words really constitutes “intelligence.” Still, the results looked far more impressive than anything on offer from the cyberneticists. And GOFAI code like ELIZA ran efficiently, even on the earliest general-purpose computers.
Many researchers and engineers didn’t care if programmed behaviors were brittle; they were busy launching a trillion-dollar industry. When AI grants stopped getting funded, they found plenty of other applications that didn’t require anything like cognitive flexibility. Computer science began to disentangle itself from AI to become a discipline in its own right, while software engineering crossed the chasm from the military-industrial complex to big business, and from there into home computers. Nobody regarded their home computer as even remotely intelligent or brain-like, though by 1977, it was easy enough to spend an hour or two typing the ELIZA program into your Altair 8800, just for laughs. 37
Meanwhile, cybernetic philosophy was going ever more meta, and was ultimately embraced (sometimes as little more than a metaphor) by the intellectually hip in fields as far-flung as government, 38 anthropology, 39 ecology, 40 urban planning, 41 sexology, 42 feminism, 43 and post-structuralist critical theory. 44 Some cybernetically inspired period pieces still make for great reading, some had the right idea, and some had significant impact in their own fields. But unlike mainstream computer science, none were accompanied by much technical progress in cybernetics itself. The concept of a “cyborg,” a cybernetically inspired superhuman fusion of man and machine (yes, usually man), went from a serious research priority at advanced defense labs to a comic-book trope. 45
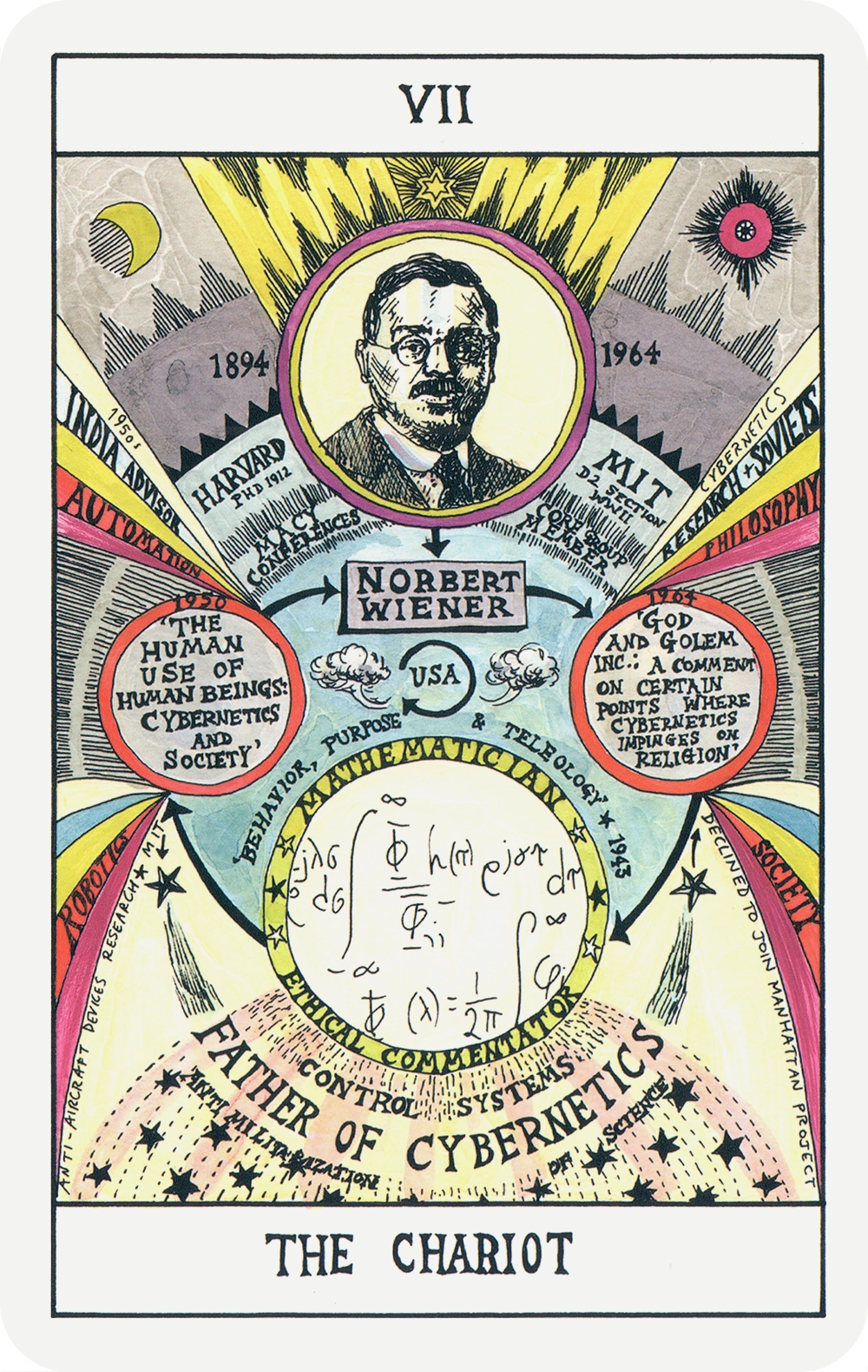
Selected cards from Suzanne Treister’s Hexen 2.0 cybernetic tarot, 2013
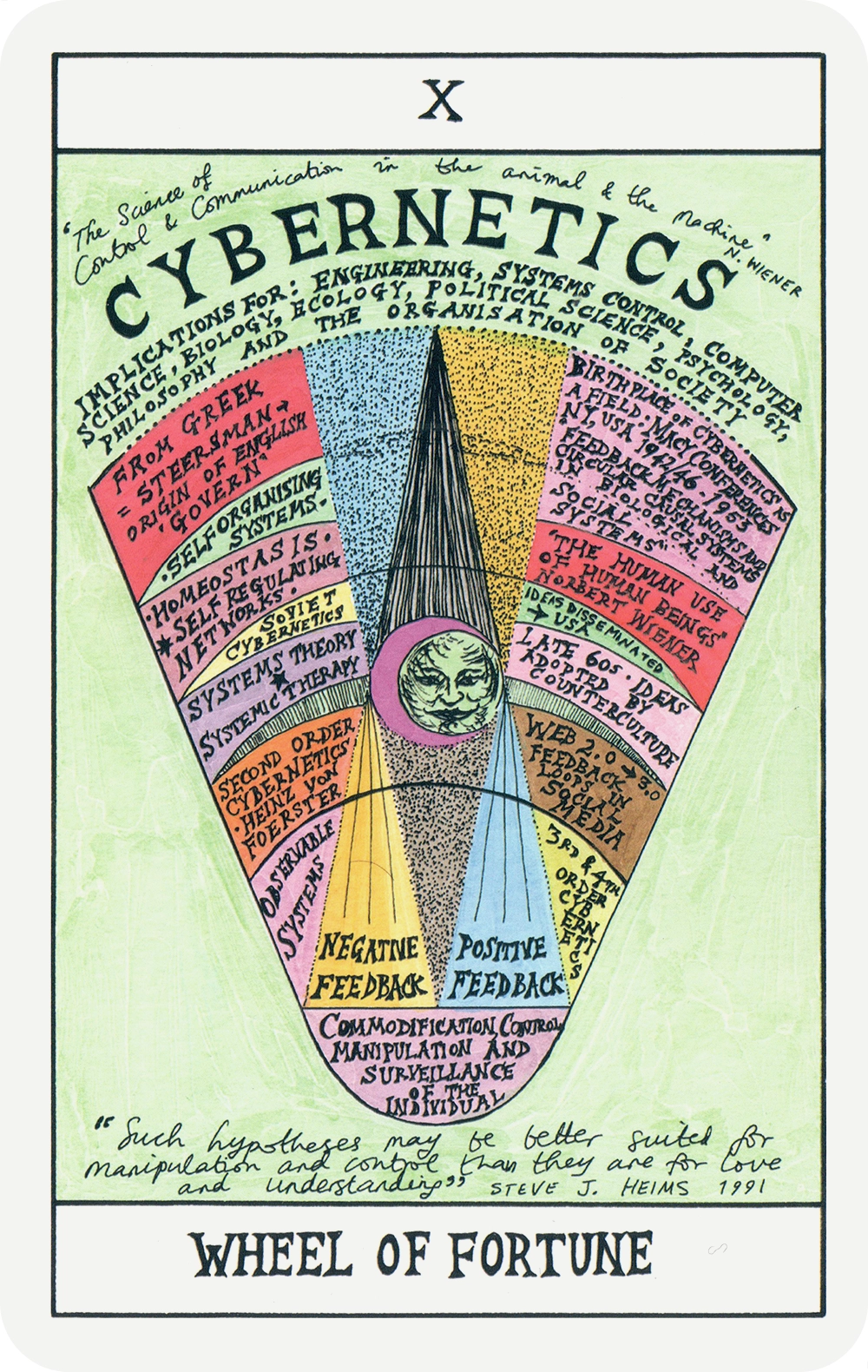
Selected cards from Suzanne Treister’s Hexen 2.0 cybernetic tarot, 2013
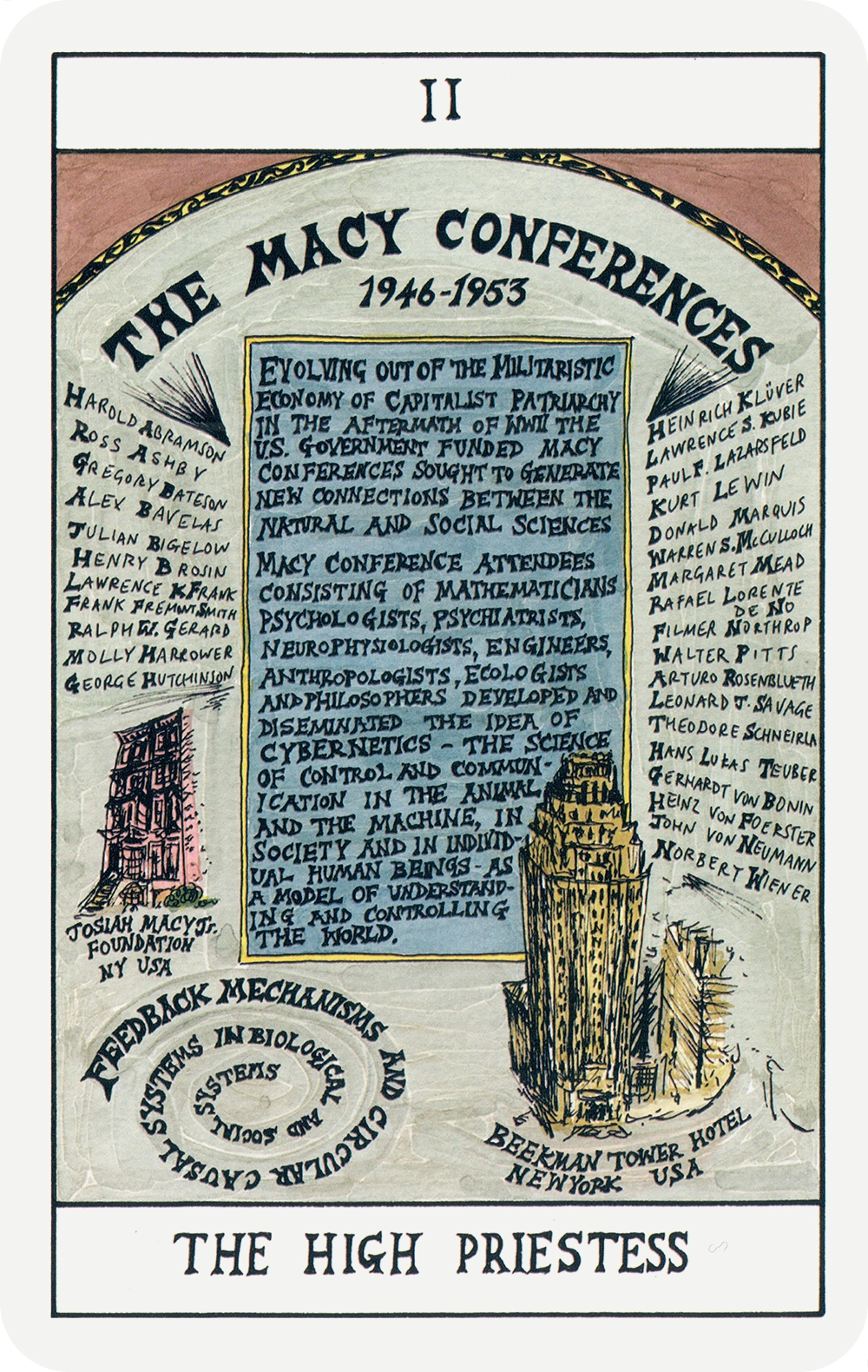
Selected cards from Suzanne Treister’s Hexen 2.0 cybernetic tarot, 2013
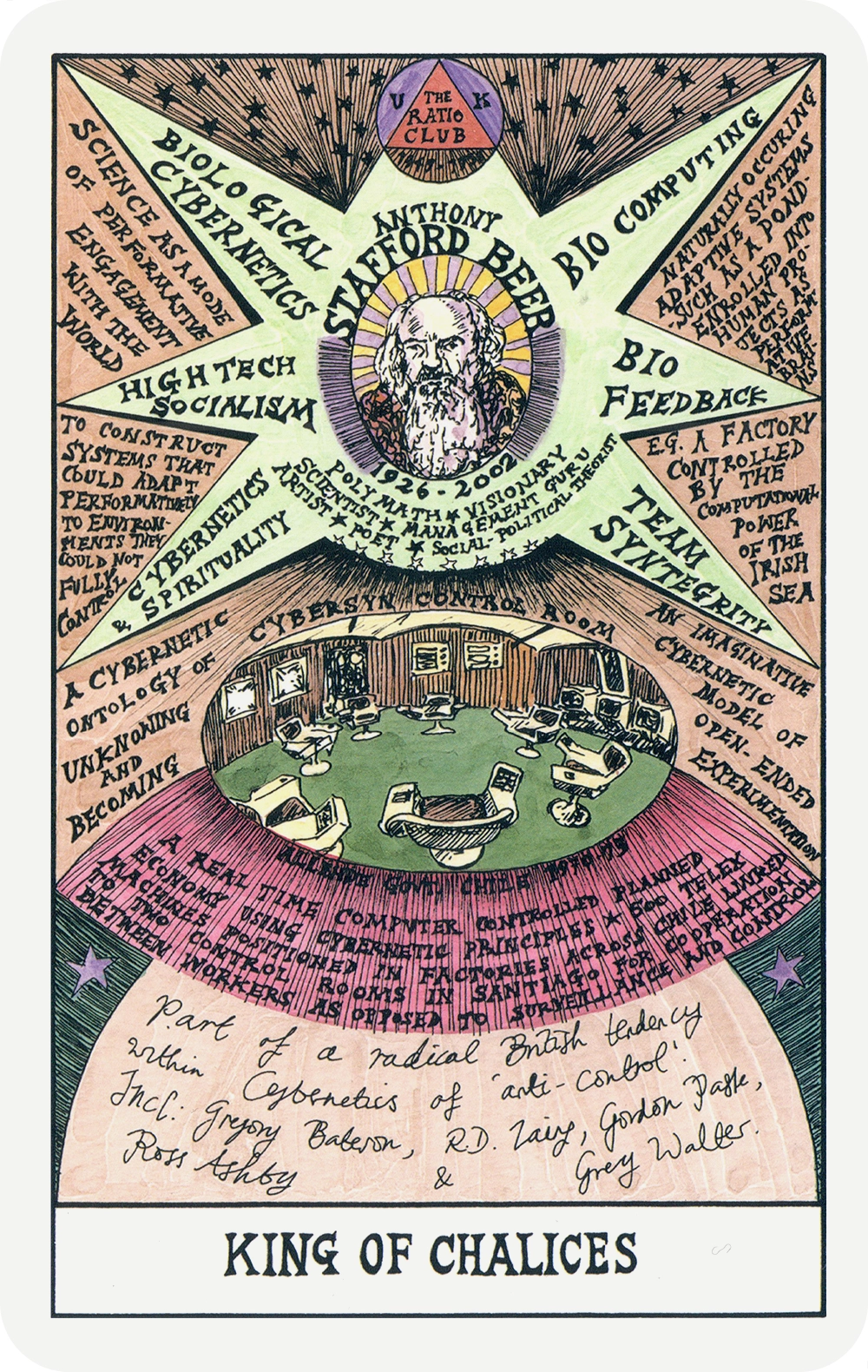
Selected cards from Suzanne Treister’s Hexen 2.0 cybernetic tarot, 2013
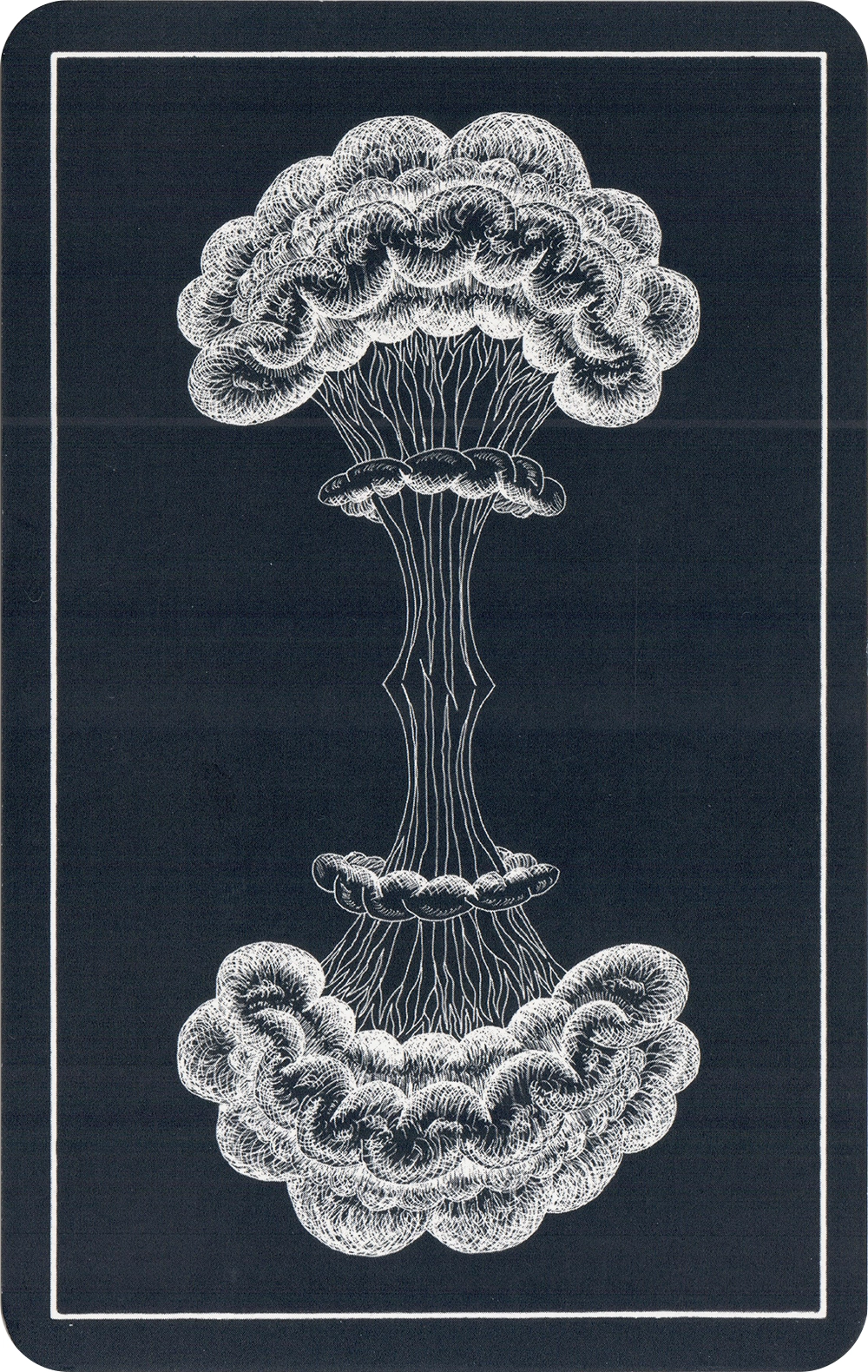
Selected cards from Suzanne Treister’s Hexen 2.0 cybernetic tarot, 2013
In other words, cybernetics began to look like a fad. Even by the mid-1960s, it had largely faded as an area of active research, though the name has survived vestigially, and somewhat randomly, in terms like “cyberspace,” “cybersecurity,” “cybercrime,” and “cyberwarfare.” 46
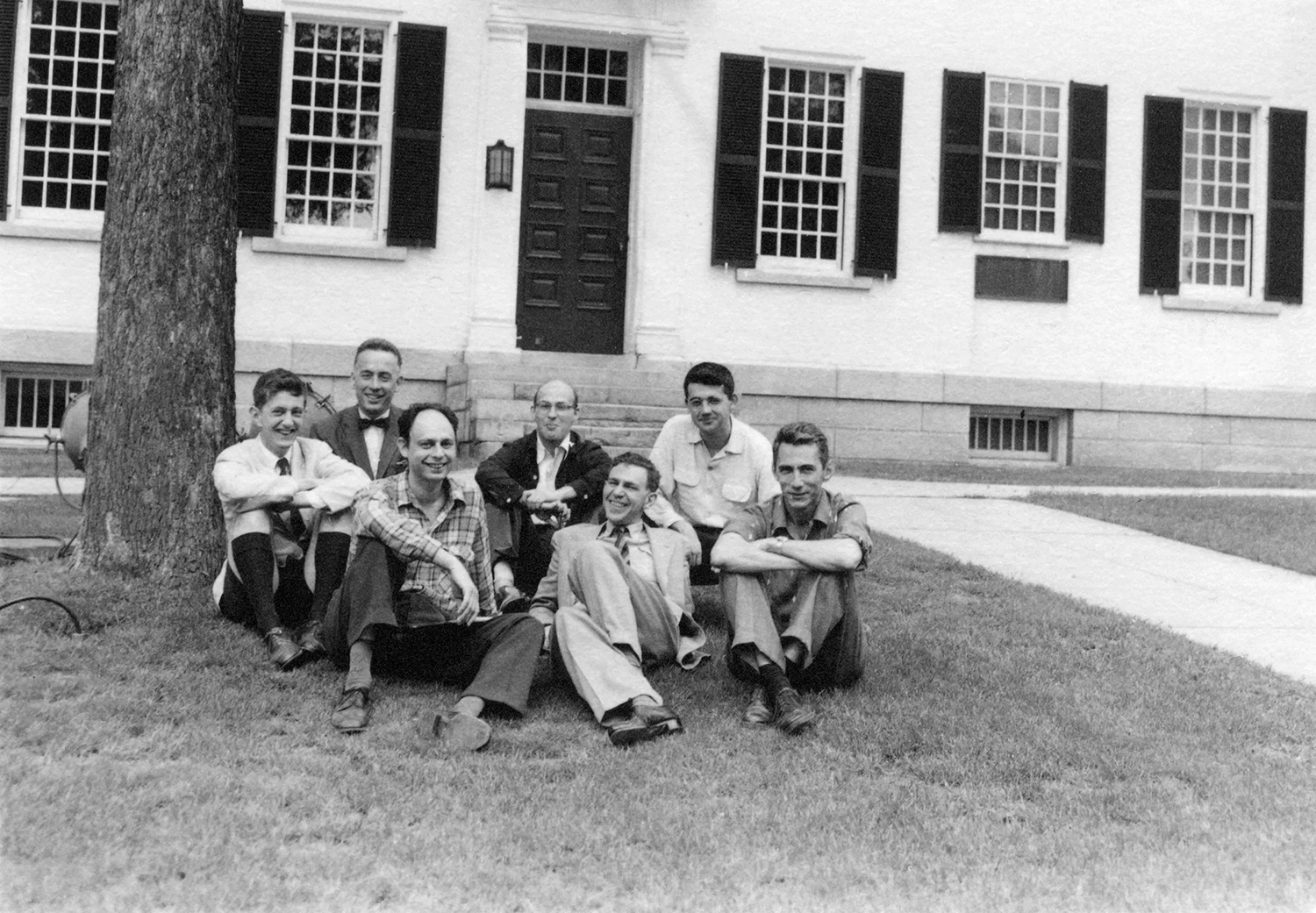
Group portrait of participants at the Dartmouth Summer Research Project on Artificial Intelligence in 1956. Back row, left to right: Oliver Selfridge, Nathaniel Rochester, Marvin Minsky, and John McCarthy. Front row: Ray Solomonoff, Peter Milner, and Claude Shannon.
Cybernetics fell victim not only to overreach, but also to the systematic efforts of its detractors. 47 In 1955, computer scientist John McCarthy coined the term “Artificial Intelligence” in the proposal for a summer workshop at Dartmouth—precisely to distinguish the new symbolic (a.k.a. GOFAI) approach from cybernetics. Despite promising “a significant advance” over the two-month period, little headway was made at the workshop proper, but its attendees, including Arthur Samuel, Allen Newell, Herbert Simon, and Marvin Minsky, became the who’s who of AI over the next several decades. Wiener was pointedly not invited. 48
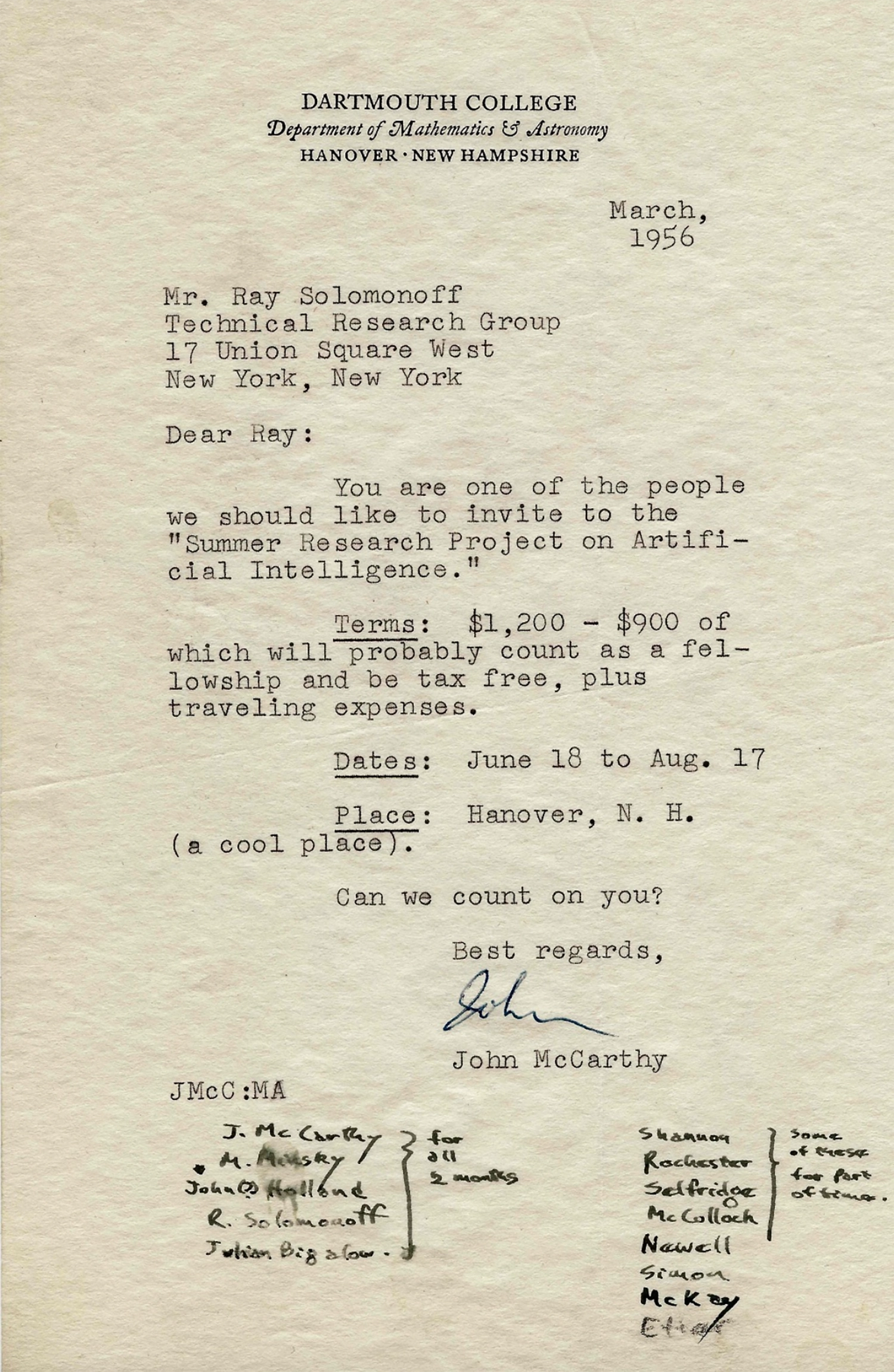
Ray Solomonoff’s March 1956 invitation to the Dartmouth Summer Research Project, from John McCarthy
It’s ironic that we continue to use the term “Artificial Intelligence,” given that today, virtually the entire field descends from the kind of work the coiners of this phrase sought to discredit—especially, per philosopher Hubert Dreyfus, “the perceptrons proposed by the group Minsky dismisses as the early cyberneticists.” 49 These were the first artificial neural nets.
How We Know Universals
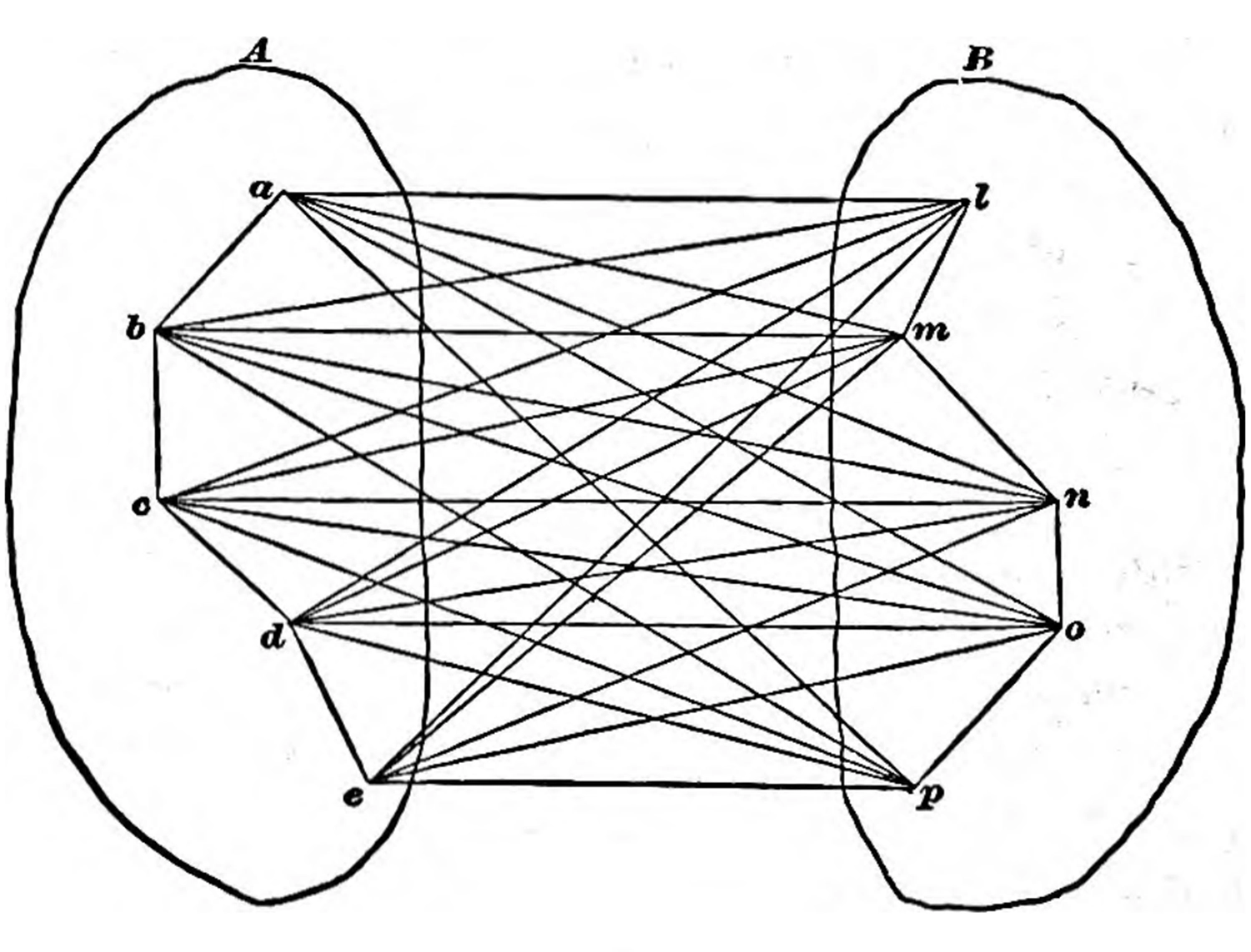
Early representation of an associative neural network from James 1890
Inspired by the brain’s physical structure, pioneering American psychologist William James (1842–1910) had envisioned something very like neural networks as far back as 1890. In his magisterial textbook, The Principles of Psychology, 50 James imagined that neural processes—the minutely complex “wires” evident in stained tissue samples from the cerebral cortex—might physically embody associations between co-occurring stimuli.
Excerpt from an experimental film by Alexis Gambis based on Santiago Ramón y Cajal’s anatomical illustrations
Shortly afterward, the great Spanish neuroanatomist Santiago Ramón y Cajal (1852–1934) concluded that this wiring is not “reticular” (meaning continuous), but consists of the branching outgrowths of individual neurons. Influenced by James, Ramón y Cajal went on to suggest that the junctions between neurons, which we now call synapses, were the sites of neuroplasticity. Associations could be learned (or unlearned) through the selective strengthening (or weakening) of synapses. 51
The idea that mental associations are central to learned behavior has its own long history. In 1897, Ivan Pavlov (1849–1936) published his classic experiments in which dogs were taught to associate food with the sound of a musical triangle. Once conditioned, they began salivating when the triangle was struck. 52
Historical footage of Ivan Pavlov’s Nobel ceremony and conditioning experiments on dogs, demonstrating salivation in response to stimuli associated with food
Learning associations is even more fundamental than behavioral experiments like this suggest. Dogs aren’t born with individual neurons that activate exclusively at the taste or smell of food, the sight of a particular person, or the sound of a triangle. Associating these multimodal events with each other is the easy part. Without being able to learn subtle, complex associations among raw stimuli, a dog would be unable to recognize a person or a ringing triangle at all.
McCulloch and Pitts formulated this problem in their 1947 paper, “How We Know Universals: The Perception of Auditory and Visual Forms.” 53 Their theory was (once again) wrong, but the question posed by the paper’s title is a good one: How do we recognize categories, or “universals”? Four years after the publication of “A Logical Calculus of the Ideas Immanent in Nervous Activity,” experiments had convinced them that neurons were not logic gates, although they did exchange excitatory and inhibitory signals. The researchers had also realized that one of the hardest computational tasks facing the brain is that of achieving “perceptual invariance”—the key to performing what we now call “classification,” “recognition,” or, more broadly, “generalization.” McCulloch and Pitts were trying to figure out how anatomically plausible neural circuits in the sensory cortex might do that.
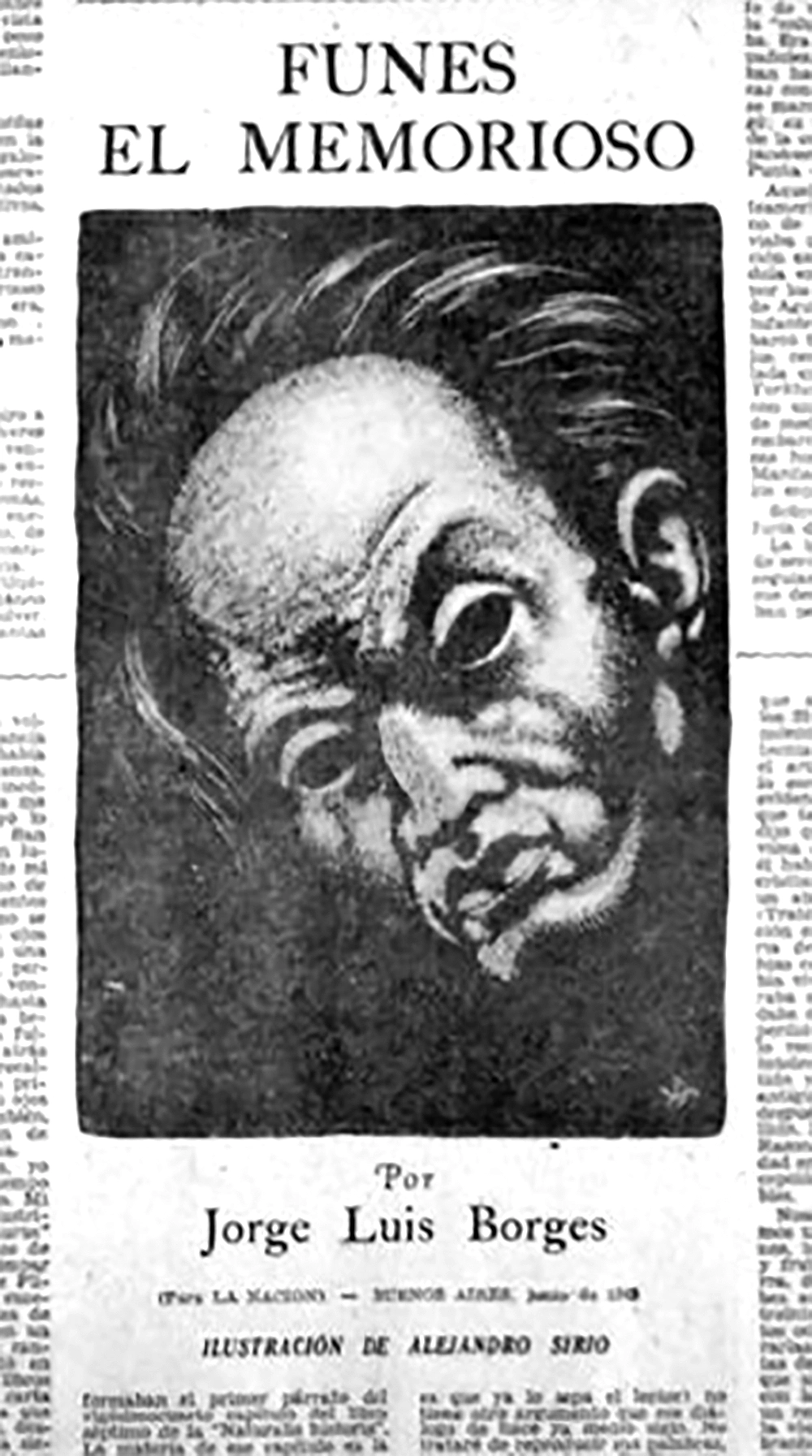
Page three of the Arts and Letters section of La Nación on 7 June 1942, where “Funes El Memorioso” was first published
Perceptual invariance is illustrated by an insightful short story, “Funes el memorioso,” published by Jorge Luis Borges in 1942. 54 In Borges’s story, a young man, Ireneo Funes, is thrown from a horse and suffers a crippling brain injury. It leaves him with a perfect memory yet robs him of the ability to generalize. After the accident, Funes can remember “not only every leaf of every tree of every wood, but also every one of the times he had perceived or imagined it”; yet he is described as “[…] almost incapable of ideas of a general, Platonic sort. Not only is it difficult for him to comprehend that the generic symbol dog embraces so many unlike individuals of diverse size and form; it bothers him that the dog at three fourteen (seen from the side) should have the same name as the dog at three fifteen (seen from the front).”
For a long time, machines fared no better. Throughout the twentieth century and well into the twenty-first, memory technologies improved exponentially, but perceptual invariance seemed to remain an unsolved problem. Attendees at the AI summer workshop in 1956 made no progress, and when those who were still alive fifty years later returned to Dartmouth for a reunion, little seemed to have changed. A computer could, like Funes, “remember” the color of every pixel in a two-hour movie, yet be unable to make any sense of those tens of billions of pixels. Hence bots could easily be foiled by visual Turing Tests as simple as “click on the images below containing a dog.” 55 The reconvening wizards, grizzled but as opinionated as ever, were of various minds about what to try next, but they all agreed that AI was a long way off. 56
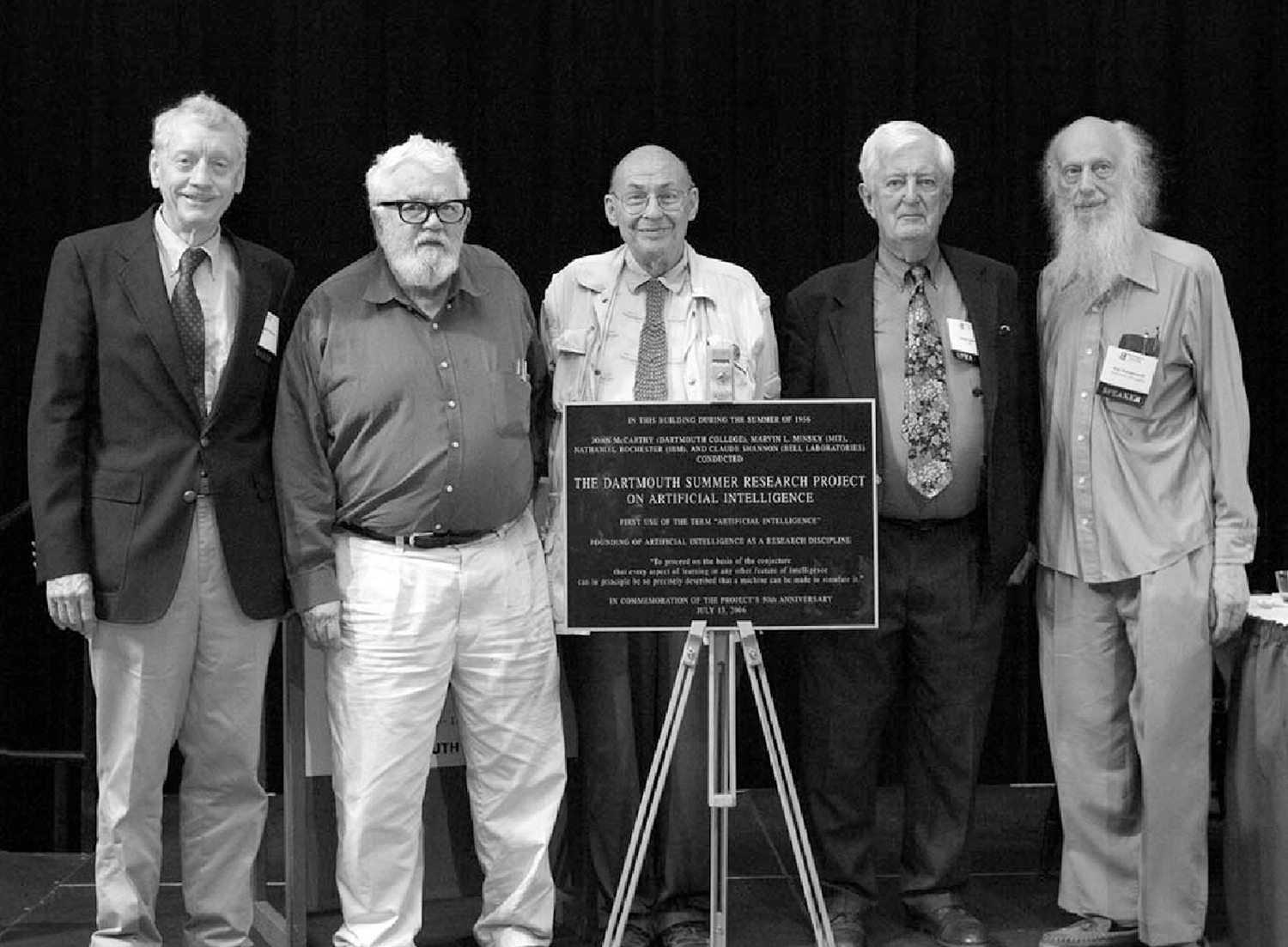
Trenchard More, John McCarthy, Marvin Minsky, Oliver Selfridge, and Ray Solomonoff, surviving attendees of the 1956 Dartmouth Summer Research Project, reconvening at Dartmouth in 2006
The year was 2006. They could not have been more wrong.
Perceptrons
The solution had lain hidden in plain sight for over a hundred years. At the turn of the twentieth century, William James and Santiago Ramón y Cajal had already accurately described how brains must learn associations. As experimenters figured out how to move beyond dissection and tissue staining to study the living brain at work, a clearer view of neural information processing began coming into focus.
In a series of papers beginning in the 1950s, neuroscientists David Hubel, Torsten Wiesel, and a rotating cast of collaborators reported on painstaking investigations of the cat and monkey visual cortex. 57 While they presented visual stimuli to animals under anesthesia, they recorded the activity of individual neurons using tungsten electrodes. They found that the “retinal ganglion cells,” which combine inputs from photoreceptors in the retina to create local visual features, are only the first stage in a visual processing hierarchy. In the brain, those features are in turn combined to form higher-level edge-like features by “simple cells,” which are themselves combined to form yet higher-level features by “complex cells,” which combine to form “hypercomplex cells”—until eventually, the features in question may be entire objects.
Hubel and Wiesel’s experiments on the cat visual system in the 1950s
Margaret Livingstone, a neuroscientist at Harvard who did postdoctoral work in Hubel’s lab, wrote, in an obituary of him in 2013, “Studying vision is fun because you see what you show the animal, and when you cannot figure a cell out, you show it everything you can think of; sometimes you find surprisingly specific things that will make a cell fire, like a bright yellow Kodak film box.” 58
Hierarchical recognition is an elegant concept: any perceptual classification task, no matter how complicated, can be built up as a combination of features, which are themselves combinations of features—turtles all the way down. This seemed to be the brain’s approach to generalization.
In 1957, Frank Rosenblatt, a young engineer at the Cornell Aeronautical Laboratory, decided to build an apparatus to do what the visual cortex does. He called it the “perceptron.” As he wrote in the introduction to his report, “[I]t should be feasible to construct an electronic or electromechanical system which will learn to recognize similarities or identities between patterns of optical, electrical, or tonal information, in a manner which may be closely analogous to the perceptual processes of a biological brain.” 59
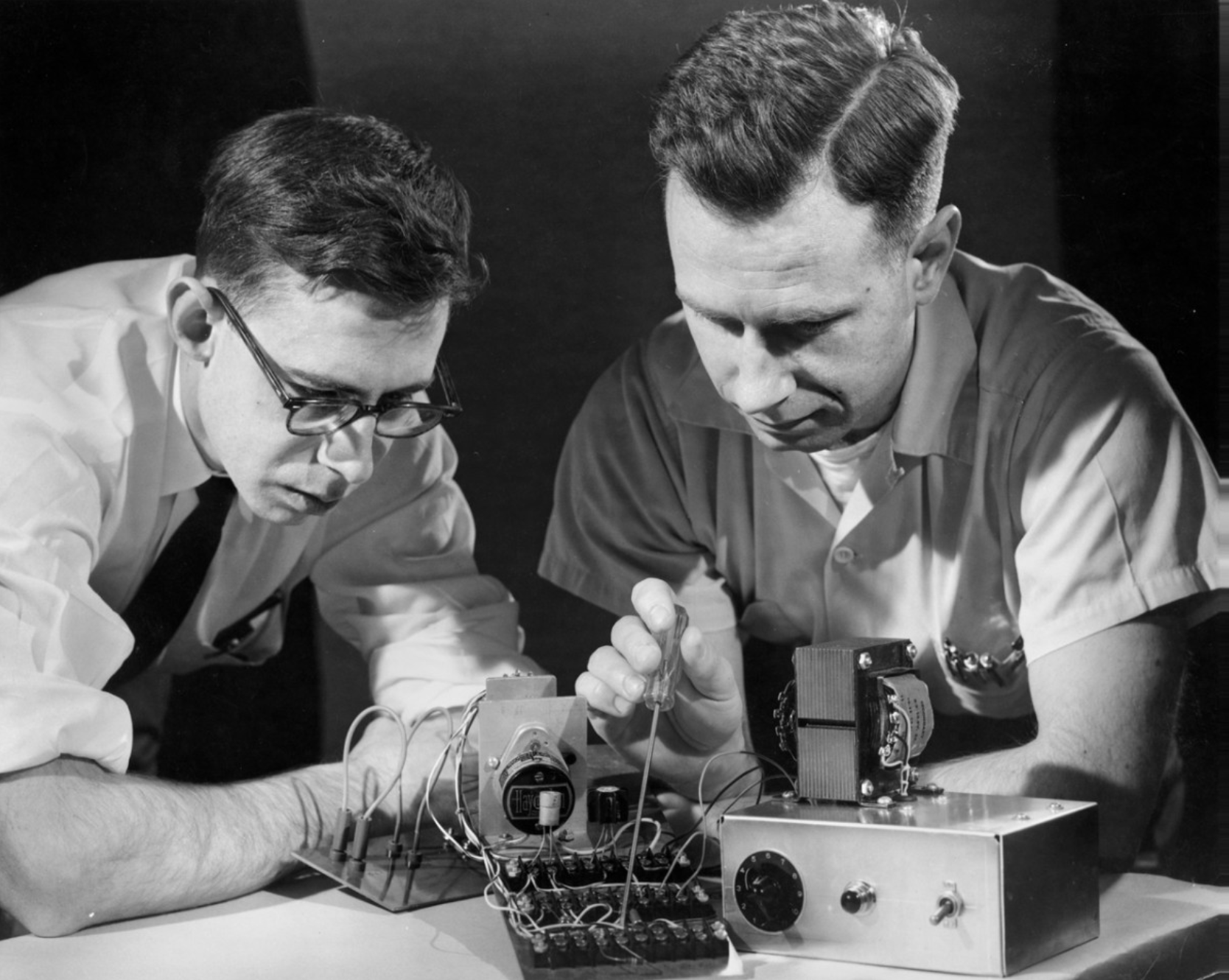
Frank Rosenblatt (left) and engineer Charles Wightman (right) work on a motorized potentiometer for the perceptron in December 1958
In a passage reminiscent of Borges, Rosenblatt noted that although “[t]he recognition of ‘similar’ forms can be carried out, to a certain extent, by analytic procedures on a […] computer […] it is hard to conceive of a general analytic program which would […] recognize the form of a man seen from any angle, and in any posture or position, without actually storing a large library of reference figures […]. In general, identities of this sort must be learned, or acquired from experience […].”
Rosenblatt’s “identities” were the same as McCulloch and Pitts’s “universals” a decade earlier. Unlike McCulloch and Pitts, though, Rosenblatt created a real, working system.
The original Mark I perceptron was a three-layer neural net. Its first layer consisted of a 20×20 array of photocells, or “sensory units,” the second had 512 “association units,” and the final layer had eight “response units.” Each layer was wired randomly to the next, with a motor-driven potentiometer (like the volume knob on a radio) modulating the strength of every connection.
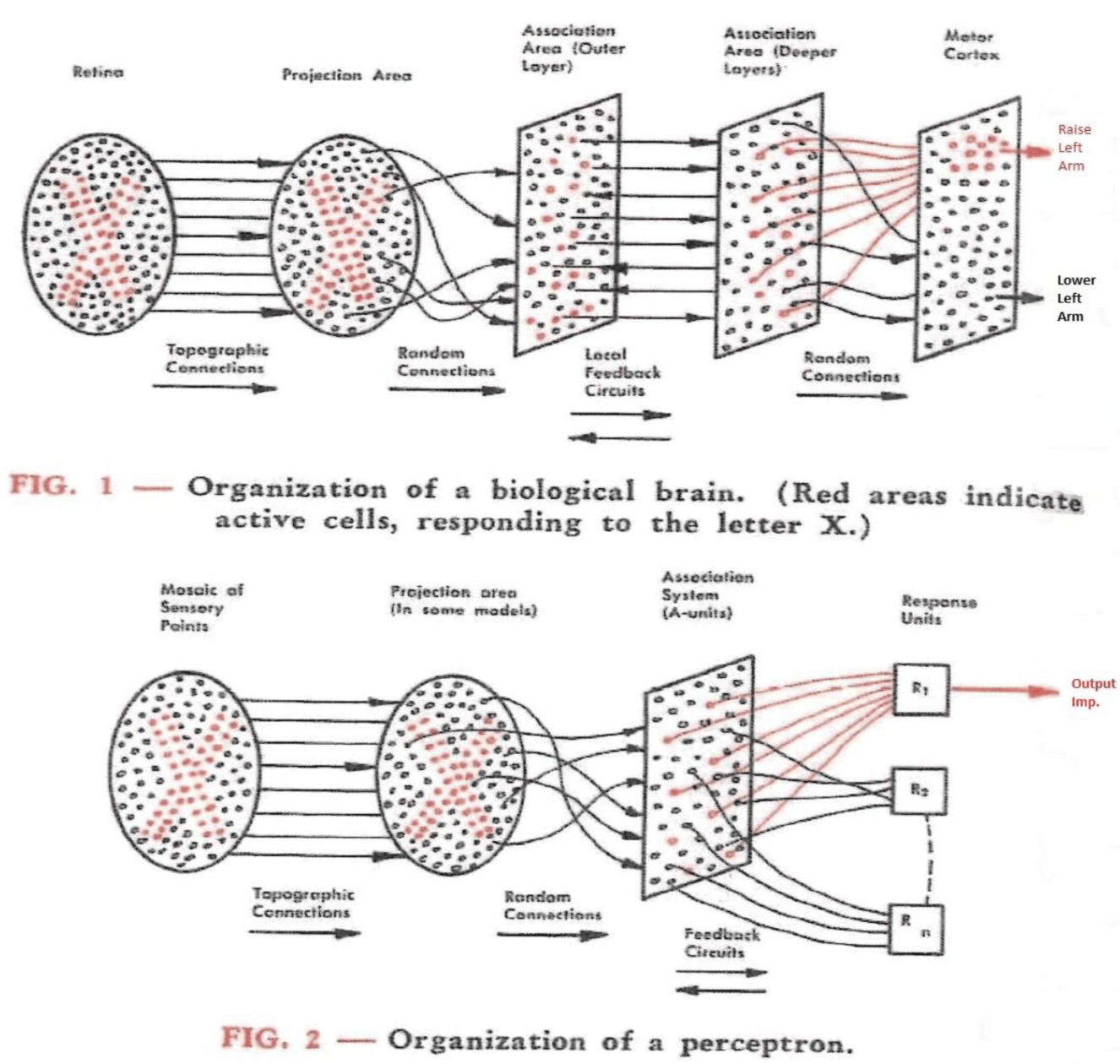
Diagram comparing the putative organization of the brain’s visual system (top) and the perceptron (bottom), from Rosenblatt 1958
The response units also implemented “lateral inhibition,” a feature commonly found in neural circuits: when one activated, it inhibited the others, which in turn fed back inhibition to any competing association units. Using a simple learning rule that exploited this feedback, Rosenblatt got the device to distinguish among simple shapes—a square, a circle, a triangle. It was a start.
More than a start: in retrospect, the perceptron was arguably the single most important advance in AI in the twentieth century. Modern neural nets, especially those most commonly used for visual recognition, are still powered by “multilayer perceptrons” (MLPs), although they use different learning rules and are typically much deeper—that is, they have many more than three layers.
A 1960s perceptron attempting to learn how to classify gender from a photo
If perceptrons worked—and did so by taking an approach so much more obviously brain-like than symbolic AI systems—then why did they lay fallow for so long? Several narratives have merit:
- Neural nets big enough to do practical work require large-scale parallel computation, which didn’t become available to most researchers until inexpensive GPUs (graphics processing units) began flooding the market, around 2006. 60 This is true; however, if, beginning in the 1960s, we had begun to seriously engineer parallel neural computers, or special-purpose hardware like the Mark I, we probably could have had powerful MLPs by the 1980s. Engineers didn’t push much in this direction because Moore’s Law advanced ceaselessly for six decades after the invention of the transistor in 1947, shrinking their size, increasing their maximum clock speed, and decreasing the power the needed to operate. The industry was eager to reap the most obvious benefits—making the whole computer smaller, faster, cheaper, and lower-power—rather than sacrificing these benefits to increase parallelism in the service of an unproven approach to computing. 61
- Large neural nets require large datasets to train, which weren’t available before the web took off. And labeled datasets, like ImageNet (which researcher Fei-Fei Li began gathering in 2006—that year again!), relied not only on internet-scale collections of images, but also on online gig workers to apply labels, like “chihuahua” and “blueberry.” All true, but let’s keep in mind that Netflix began sending subscribers digital movies by DVD nearly a decade earlier, and a modest collection of DVDs contains far more imagery than ImageNet. There was no shortage of pixels. We also now know that unsupervised learning works well, rendering extensive labeling unnecessary (as will be discussed in the next chapter). This would likely have been discovered decades earlier had neural-net research remained mainstream in the latter half of the twentieth century.
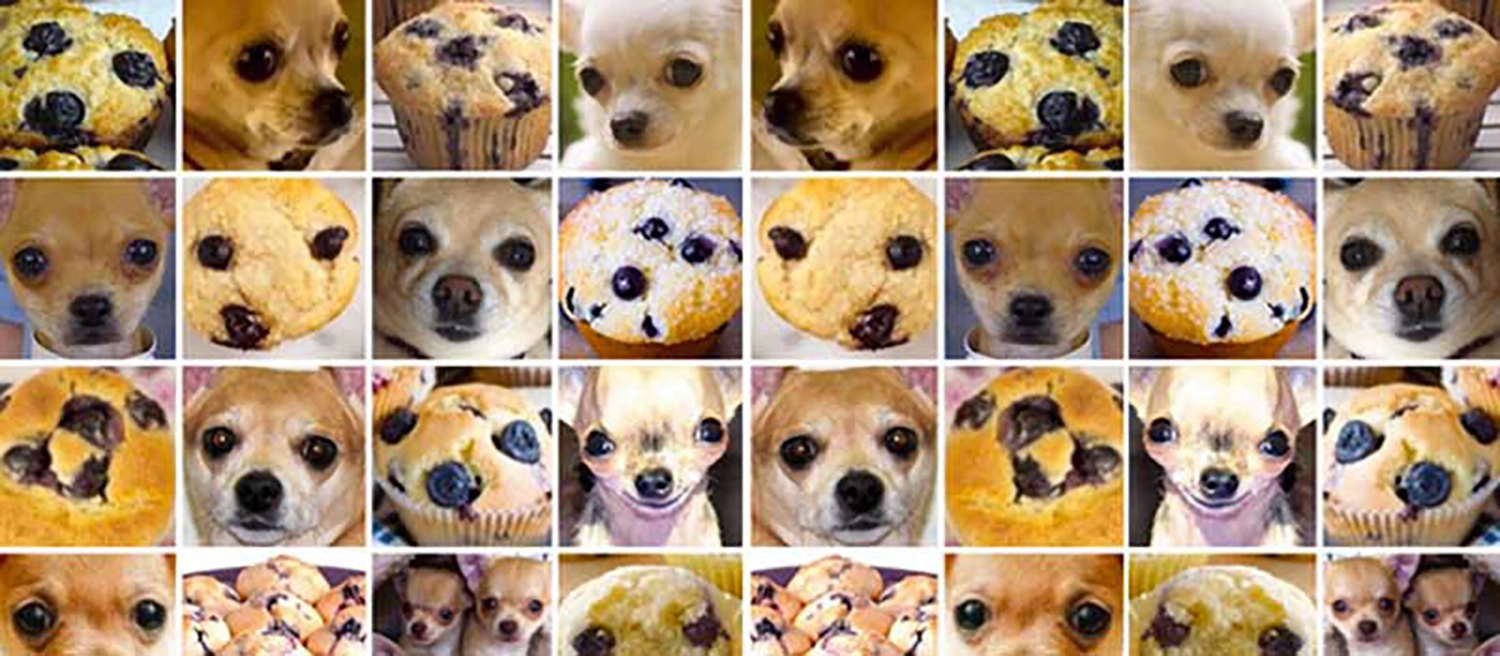
In 2016, grids of images depicting either chihuahuas or blueberry muffins began circulating on the internet; initially, their visual similarity posed a challenge for CNNs
- Rosenblatt’s original perceptron-training algorithm wasn’t powerful enough to work for complex datasets or deep neural nets; the suite of tricks required to do so has only been perfected in recent years. The most important of these tricks, though—the “backpropagation” algorithm, allowing the weights of synapses, or “synaptic weights,” in an arbitrarily deep neural net to be adjusted to minimize the output error—had already been worked out by 1970, and was repeatedly reinvented by other researchers over the years. 62 Like any technology, dedicated tinkering is required to get the details right. For many years, there simply weren’t enough dedicated tinkerers working on it, in part because—
- In 1969, Marvin Minsky and an MIT collaborator, Seymour Papert, published a highly cited book entitled Perceptrons. 63 Although dedicated to Rosenblatt, the book was a hatchet job, implying that perceptrons could never approximate a number of simple mathematical functions, and therefore perceptrons were inherently far weaker, computationally, than GOFAI algorithms. The book contained mathematical proofs of these assertions, but the proofs only applied to simplified two-layer models. It can be shown that with three or more layers (as even the Mark I perceptron had) any continuous function can be approximated, 64 as Rosenblatt himself appears to have intuited. 65
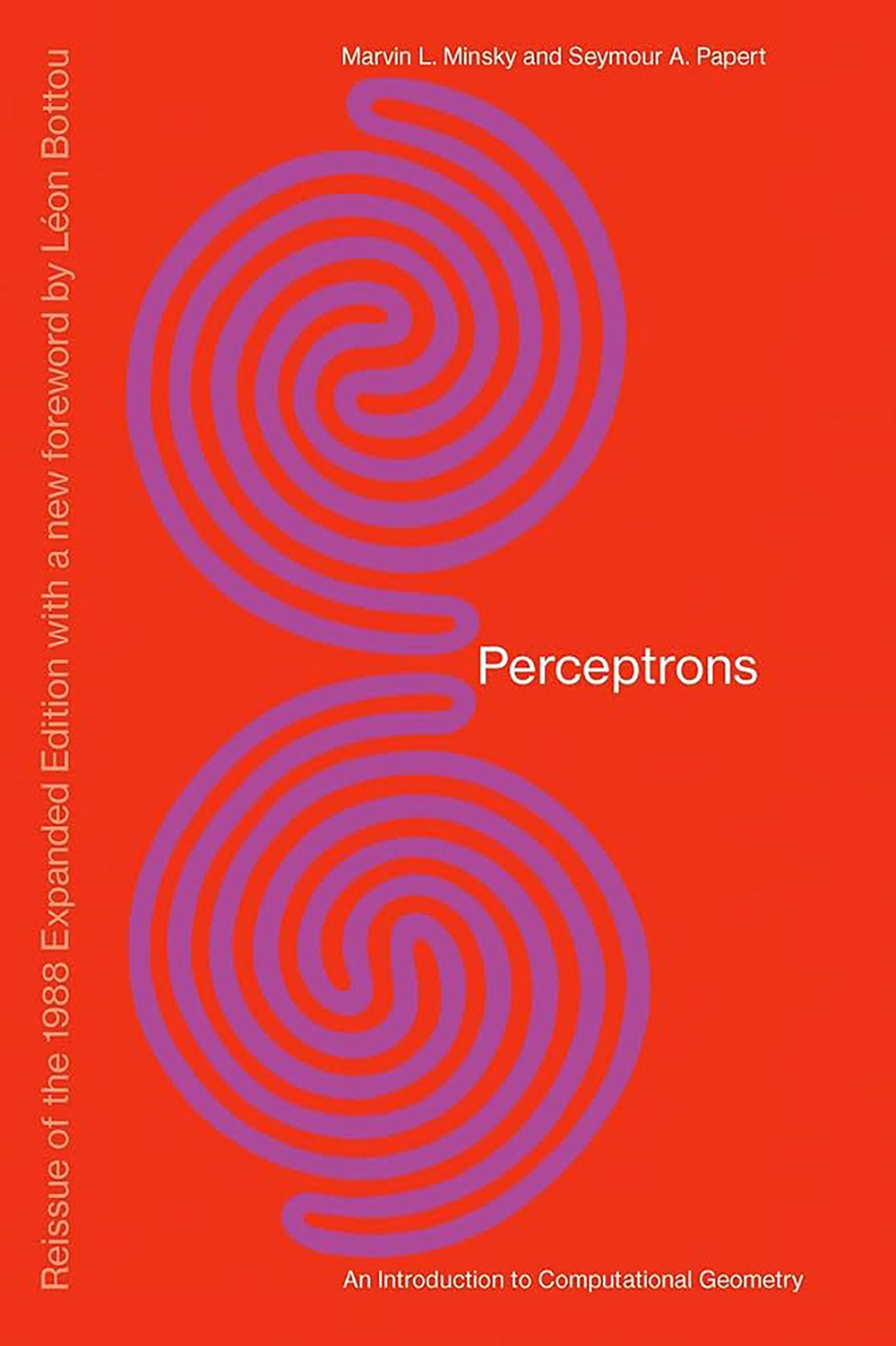
The cover of Perceptrons, by Minsky and Papert. (1972 edition, ©MIT. All rights reserved.) The authors pointed out that a perceptron can’t distinguish certain global visual features, such as the difference between the upper figure, which consists of a single purple loop, and the lower figure, which consists of two purple loops. Interestingly, a person can’t do it at a glance either.
Despite the protests of Rosenblatt and fellow travelers, the Perceptrons book was highly effective in discouraging “connectionism,” as neural net research was then called, for decades, diverting mainstream attention instead to the GOFAI approaches favored by Minsky, Papert, and their colleagues. In 1988, with interest in neural nets rising once again just as we entered a final Good Old-Fashioned AI winter, Minsky and Papert reissued Perceptrons. In a new prologue and epilogue, the updated edition doubled down on their original critique, claiming that “little of significance has happened in this field” and that, even by 1969, “progress had already come to a virtual halt because of the lack of adequate basic theories.”
These claims seem bizarre, if not disingenuous. Just a year before the second edition of Perceptrons, computational neuroscientists Terry Sejnowski and Charles Rosenberg had trained a neural net, NETtalk, to pronounce English text. They showed not only that it could effectively master this notoriously unruly (that is, non-GOFAI-friendly) problem, but that its performance exhibited many human-like characteristics. 66
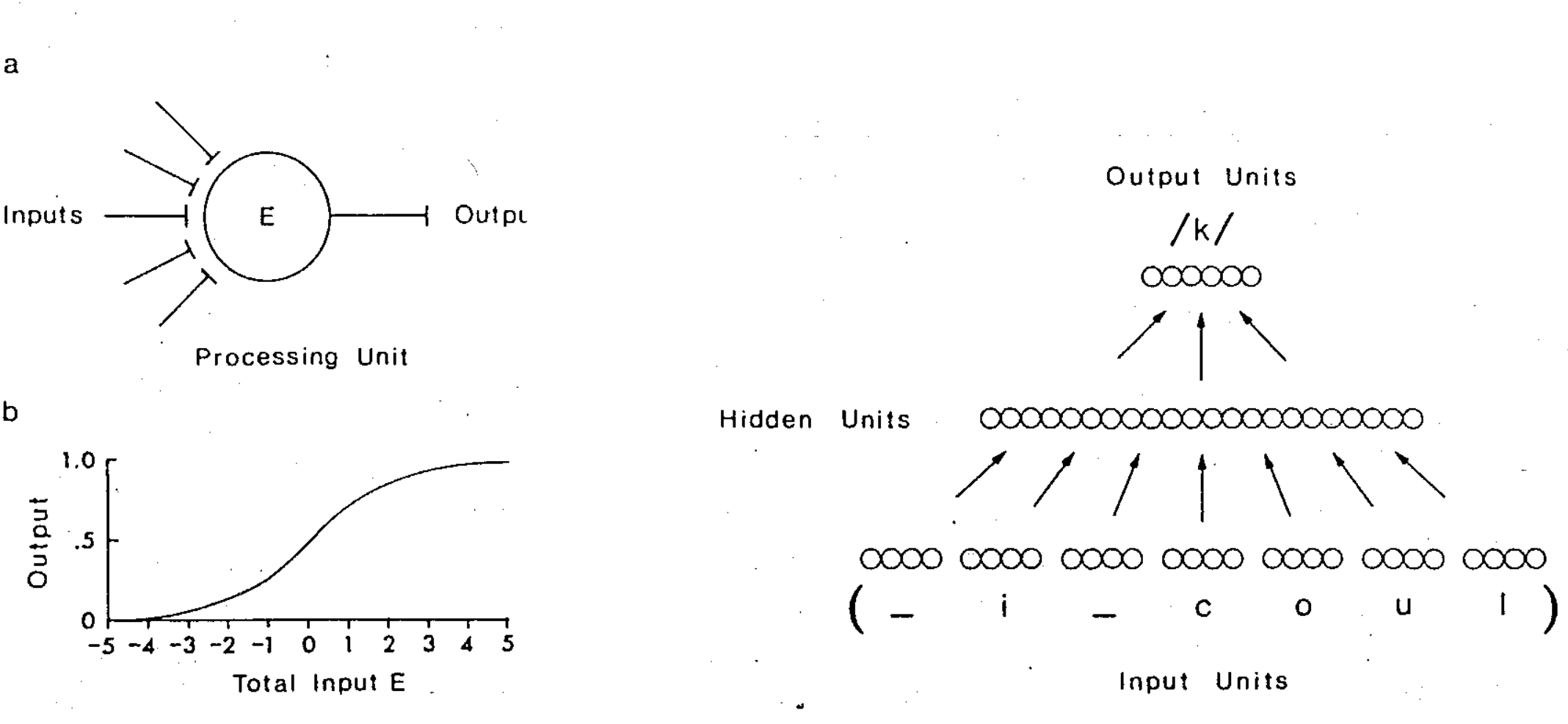
Design of the NETtalk artificial neural network for translating English letter sequences into phonemes, Sejnowski and Rosenblatt 1986
Minsky and Papert’s objections to connectionism make some sense, though, in light of the theoretical grounding they had been hoping AI might offer. They were uninterested in the rapid advances taking place in computational neuroscience, machine learning, or general function approximation. Instead, they focused on mathematical theories of knowledge representation, manipulation, and formal proof in the tradition of Leibniz. This required operating at the level of abstract concepts, more like the “psychons” McCulloch and Pitts had speculated about in 1943 (but that neuroscience had shortly thereafter abandoned, failing to find any evidence of them in the brain). Per Minsky and Papert: “perceptrons had no way to represent the knowledge required for solving certain problems. […] No machine can learn to recognize X unless it possesses, at least potentially, some scheme for representing X.”
They wanted, in other words, for knowledge to be represented logically, in terms of clear taxonomies and semantic relationships—just as Leibniz had imagined. And such logical representations would, they believed, need to look like Boolean, yes-or-no variables somewhere specific in the brain. Without such “schematized” and “localized” knowledge representations, Minsky and Papert did not believe that higher-order rationality, including causal reasoning, self-reflection, or even consciousness, would be possible: “[W]e expect distributed representations 67 to tend to produce systems with only limited abilities to reflect accurately on how they do what they do. Thinking about thinking, we maintain, requires the use of representations that are localized enough that they can be dissected and rearranged. […] [D]istributed representations […] must entail a heavy price; surely, many of them must become ‘conceptual dead ends.’” 68
Unlike the book’s proofs, these assertions were mere intuitions. To many, they seemed reasonable at the time. Adherents of the GOFAI school of thought, including many trained in linguistics (which is largely concerned with schematized knowledge representations) continue to argue this position today, though it seems increasingly disconnected from reality given what modern neural nets can do, and how they do it. 69
Deep Learning
Training multilayer perceptrons is difficult both because of the dependencies between layers (fiddling with a synaptic weight affects everything downstream) and because of the dramatically increased sizes of these models. To get a sense of this, consider that a two-layer “fully connected” perceptron classifying a 32×32 pixel image as one of ten digits already has (32×32)×10=10,240 synapses—that is, a synapse connecting every pixel with each of the ten output neurons. If we add in a single additional 32×32 layer of neurons between the input layer and the output layer, we now have (32×32)×(32×32) + (32×32)×10=1,058,816 synapses. Each of these synaptic weights is a model parameter that must be learned from data, and, roughly speaking, fitting a hundred times more parameters requires a hundred times more data. 70
Over time, though, researchers found ways around these problems. The story of modern “deep learning” is just the story of these tricks accumulating over time, with compounding gains and accelerating progress as the field finally attracted serious time and investment. Here are a few representative advances. A complete list would be much longer: 71
- Sparse connectivity, dating all the way back to Rosenblatt’s perceptron, can greatly reduce the number of weights compared to a “fully connected” layer as sketched above; this makes sparse networks faster to train and more reliable. In the 1980s, Yann LeCun, John Denker, and Sara Solla, then at Bell Labs, figured out how to prune unneeded weights in a more principled way. 72
- Deep learning itself is a form of sparse connectivity, when you consider that a truly fully connected neural network wouldn’t have layers at all; every neuron would be connected to every other neuron. In a multilayer perceptron, though, a neuron is only connected to neurons in the previous layer and the next layer. Hence, the deeper a network is, the sparser its connectivity compared to a fully connected network with the same number of neurons. In the mid-2010s, researchers pushed this form of sparsity to extremes, exploring architectures with up to a thousand layers. 73
- “Convolutional layers,” first conceived by AI researcher Kunihiko Fukushima and further developed by LeCun and collaborators, 74 implement yet another form of sparse connectivity by wiring a neuron only to a small neighborhood of neurons in the previous layer, rather than to all of them; furthermore, an entire “channel” of neurons share a common weight pattern within this local area, making the number of parameters to train far smaller than the number of connections.
- The backpropagation algorithm, as mentioned earlier, allows minimization of an error, or “loss function,” to happen reliably through many layers of neurons. It involves using calculus to compute the total downstream effect of fiddling with any given synaptic weight. Virtually all machine learning today uses backpropagation.
- The “softmax” function, 75 applied to a layer of neurons, implements something analogous to lateral inhibition, picking out the maximum activation and enhancing it while suppressing competing neurons, while forcing the layer’s total activity to sum to one. Softmax was originally used only for the output layer of classifier nets, which are trained to be “one-hot” (a single neuron on, all the others off). However, the suppression of competing activations isn’t complete (hence the “soft”), allowing learning through backpropagation to work.
- “Max pooling” 76 can discard unneeded resolution, for instance reducing a 32×32 layer of features to a 16×16 layer by picking only the largest values within each 2×2 region to pass on. This works because the presence of complex features is often more important than their precise location.
- Datasets can be “augmented” 77 by shifting, stretching, and rotating training examples, then using these distorted copies as additional training data. This helps strengthen the neural net’s perceptual invariance.
Kunihiko Fukushima and colleagues present their 1979 Neocognitron
It’s significant that researchers working at the intersection of computer science and neuroscience pioneered many of these techniques. In recent years, this confluence of disciplines has been called “NeuroAI,” and a number of conferences and workshops have been convened under that banner.
While the name is new, the phenomenon is not. 78 From the beginning, machine learning and neuroscience have been continuous with one another, as we’ve seen. For many years, the scientific value of this interdisciplinary work was greater than its technological value, so it was often referred to as “computational neuroscience.” With the technological side now ascendant, it’s unsurprising to see the ordering reversed with “NeuroAI.” But the conference attendees are largely the same. And while no practical machine-learning trick faithfully models any specific process or circuit in the brain, many of the tricks are clearly biologically inspired, just as the original perceptron was. 79
Using such tricks in combination, researchers fully solved handwritten single-digit visual recognition in the 1990s, 80 and moved on to increasingly difficult visual-recognition problems over the following two decades: objects, clothes, places, plants and animals, natural scenes, faces, and ultimately nearly everything one might encounter while browsing through the photos on a phone. 81 Although they were developed mostly for the visual modality, convolutional nets also work well in many other domains, from auditory recognition 82 to weather prediction. 83 Surprisingly, they even work for classifying text, 84 but we’ll hold off on exploring neural nets for language until chapter 8.
Closing the Loop
Before moving on, let’s consider neural networks through the cybernetic lens. On one hand, the perceptron did generate discrete symbolic output—per Rosenblatt’s 1957 report, “inhibitory feedback connections guarantee that only one response out of a mutually exclusive set can be triggered at one time.… [Response] units thus [act] like a multi-stable flip-flop.” 85 (In modern neural nets, softmax layers serve the same purpose.)
Still, GOFAI partisans like Minsky and Papert understood that Rosenblatt was working squarely within the cybernetic tradition, and, indeed, the response layer and learning rule only approximated digital logic in the output through the use of analog negative-feedback loops. The system learned by example, and relied on this analog quality to do so. Neural representations within the network were distributed and continuous, not localized or symbolic. Synaptic weights were continuous, and the perceptron as a whole evaluated a nonlinear continuous function specified by those weights.
Rosenblatt’s perceptron made use of randomness too, both in the wiring and in the learning process: “The penalty that we pay for the use of statistical principles in the design of the system is a probability that we may get a wrong response in any particular case…” 86 The perceptron worked precisely because it was far from the world of cleanly represented knowledge and exact logical inference.
Let’s boil down the main advance Rosenblatt and his successors made over Wiener: while Wiener’s “black box functions” were expressed in terms of “series expansions,” the function evaluated by a perceptron is instead specified by a hierarchy of synaptic weights. A series expansion is a weighted sum of increasingly high-order mathematical terms, typically truncated after only a few terms. For the kinds of simple cybernetic systems Wiener and colleagues were able to build (like thermostats, or, eventually, antiaircraft-fire controllers), a single term, representing a linear approximation, might suffice. Not so for a multilayer perceptron capable of achieving visual perceptual invariance.
The problem isn’t theoretical, but practical. At bottom, a function is a function. Both neural nets and series expansions are “universal function approximators,” meaning that either one can approximate any function. 87 However, Wiener’s more formal approach would have required vast numbers of parameters to go beyond the linear or first-order regime. Realistic nonlinearities (say, for object classification) couldn’t, in practice, be learned from a limited number of training examples. By dispensing with this kind of math and simply wiring together simplified neurons in multiple layers, Rosenblatt had stumbled onto a far more learnable way of representing the kinds of functions needed to transform images, sounds, and other natural stimuli into invariant classifications. One could attribute that to luck, intuition, or both, but, at some level, something like it had to work, or our brains would all be like that of poor Funes, el memorioso.
Why perceptrons are so “learnable” relative to more traditional function approximators is not yet fully understood. 88 Recent analyses suggest that it may have to do with a “compositional prior,” meaning a bias 89 toward learning functions that can be defined in terms of a hierarchical composition of simpler functions. This kind of prior appears to be useful for all sorts of cognitive tasks and generalizations, not just vision. 90 (Intriguingly, the symbiogenetic view of evolution described in chapter 1 can also be understood as a learning algorithm that favors hierarchical compositions of functions.)
Although the perceptron is cybernetic in spirit, it doesn’t qualify as a complete cybernetic system, at least not as usually deployed. An image classifier has no obvious agency. It doesn’t act, it just is, like any other mathematical function.
One notable exception, though, is a 2016 paper from researchers at Nvidia: “End to End Learning for Self-Driving Cars,” 91 describing the DAVE-2 system (successor to DAVE, an earlier “DARPA Autonomous Vehicle”). The authors trained a convolutional neural net to “map raw pixels from a single front-facing camera directly to steering commands.” 92 Their abstract continues, “This end-to-end approach proved surprisingly powerful. With minimum training data from humans the system learns to drive in traffic on local roads with or without lane markings and on highways. It also operates in areas with unclear visual guidance such as in parking lots and on unpaved roads.”
Demo of the DAVE-2 self-driving car, 2016
DAVE-2 appears to be a remarkably pure realization of Wiener’s cybernetic vision, courtesy of the universal function-approximating power of a convolutional net. One can think of it as a much more sophisticated version of the Palomilla, with real eyes instead of photocells and twenty-seven million synapses in place of a couple of vacuum tubes. (For rough comparison, a honeybee probably has about a billion synapses and a mouse has about a trillion, though biological synapses are not interchangeable with model parameters.)
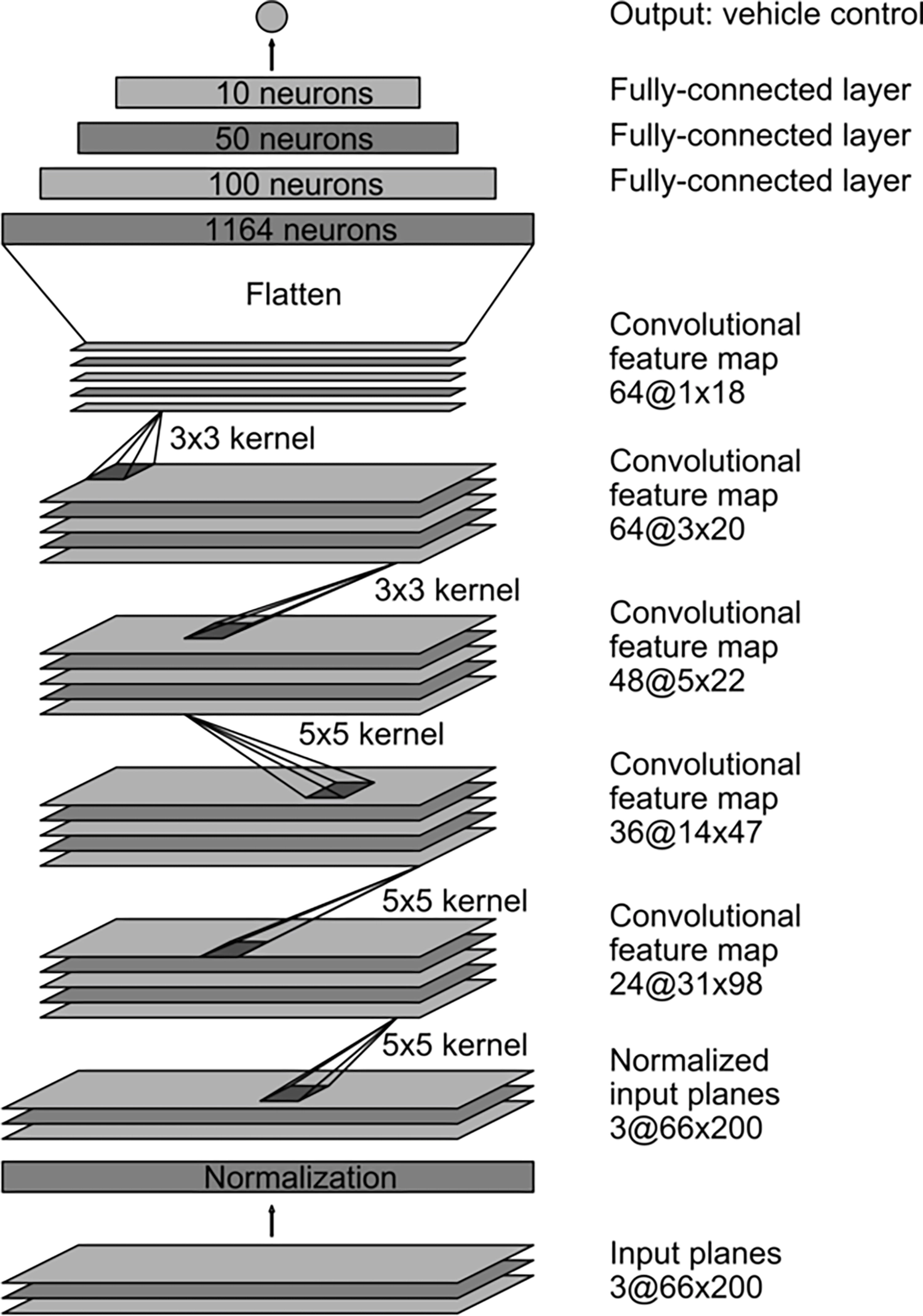
Architecture of the DAVE-2 convolutional neural net for autonomous driving, from Bojarski et al. 2016
The nonlinearity of DAVE-2’s learned function allows for rich, contextually dependent behavior without explicit “if/then/else” logic; lane markings, guard rails, and other cars influence driving in all the ways one would hope. Although the network’s only output is a steering wheel angle, if we were to perform “neuroscience” on this network after training, we would undoubtedly find neurons that respond specifically and selectively to all such objects, much like the visual-system neurons Livingstone recorded from during her work in Hubel’s lab.
This picture is still missing important elements, though. Notably, the input to the convolutional net is only a single frame of video, and the output only controls steering, not gas or brakes. It involves no prediction of the future. Since the network is run at thirty frames per second, vehicle speed is kept steady by a human copilot, and steering is forced to be reasonably smooth by mechanical inertia, the system undoubtedly feels dynamic, but the learned model has no dynamics. Nothing like planning or memory is possible here, only instantaneous reflex-like response, fully determined by prior offline training. Neither is the model modeling itself in any way; its umwelt is purely external, just a view of the road at that moment in time.
Rosenblatt’s original (and cybernetically informed) vision was considerably broader. He emphasized that what we now simply call the “perceptron” was in fact only a “momentary stimulus photoperceptron, […] the most elementary device which could be built to demonstrate the general principles of this type of system.” Not only could other sensory modalities also be imagined, but “temporal pattern perceptrons” would also have the ability to “remember temporal sequences of events, rather than transient momentary images, such as would be obtained from a collection of isolated frames cut from a strip of movie film.” 93
Most of this remained on the drawing board, but, tellingly, even the Mark I momentary stimulus photoperceptron had been implemented as a physical device with real dynamics, able to learn by adjusting its parameters based on feedback at any time. Today’s neural nets generally don’t share this property. Even if they act as controllers in a feedback loop, as in a self-driving car, they do not alter themselves in a feedback loop during operation, the way we do. Instead, they are trained offline on static data using batch processing, much the way ENIAC and the other early computers crunched away on big problems overnight (or for many nights).
Thus, the field we call “machine learning” still doesn’t generally produce systems that learn, but only systems that act. It may be hard to consider something “alive” if nothing it experiences can durably affect it, and, for many, calling something “intelligent” may also be hard if it can’t learn from those experiences. 94 In these crucial respects, we’re still working toward the ambitious vision laid out by Wiener, Rosenblatt, and the other early cyberneticists in the 1940s and ’50s.
McDonald, Rice, and Desai 2016 ↩.
D. T. Schultz et al. 2023 ↩.
Northcutt 2012 ↩.
More correctly, matter-energy is conserved, but, on Earth, outside nuclear power plants and atomic bombs, energy and matter are individually conserved, or near enough.
Most plants, of course, don’t eat other life. Their source of free energy is the stream of low entropy photons emanating from our nearest star. The Earth as a whole re-radiates higher-entropy photons back into space, maintaining an energetic and entropic balance—although the greenhouse effect, by diminishing the amount of re-radiation that can escape into space, is now upsetting this balance, resulting in rising entropy on Earth.
Per the brief discussion of competence without comprehension in “The Umwelt Within,” chapter 2, this does not necessarily imply that any such modeling is conscious. As we’ll explore in “What It Is Like to Be,” chapter 6, what we think of as consciousness is modeling your own model, not merely having (that is, acting in accordance with) a model.
Babbage 1864 ↩.
They were: Kay McNulty, Betty Snyder, Marlyn Wescoff, Ruth Lichterman, Betty Jean Jennings, and Fran Bilas. Fritz 1996; Light 1999 ↩, ↩.
Typically of “firsts,” there are other contenders. Working in relative isolation, Konrad Zuse, a German civil engineer, completed his electromechanical Z3 computer in 1941. Zuse’s project was also motivated by war; his earlier S1 and S2 were special-purpose machines for computing aerodynamic corrections to the wings of radio-controlled flying bombs, and after 1939 he was funded by the Nazi government. While Zuse didn’t design the Z3 with Turing completeness in mind, and it didn’t natively support conditional jumps, it can be programmed cleverly to simulate them. This arguably gives the Z3 priority over the ENIAC, per Rojas 1998 ↩.
Fitzpatrick 1998 ↩.
IBM 2007 ↩.
Renting the 701 in 1952 cost between $12,000 and $18,000 per month. IBM 2007 ↩.
Waldrop 2018 ↩.
De Monchaux 2011 ↩.
Though in 1834 André-Marie Ampère had anticipated both the concept and the name in his Essay on the Philosophy of Science, in which he wrote that a future science, “cybernétique,” should encompass “that body of theory […] concerned with the underlying processes that direct the course of organizations of all kinds.” Ampère 1834; G. Dyson 1998; Rid 2016 ↩, ↩, ↩.
Mindell 2002 ↩.
Galison 1994 ↩.
Nakamura and Kobayashi 2021 ↩
Mischiati et al. 2015 ↩.
When a patient with “intention tremor,” caused by cerebellar damage, reaches for an object, they tend to overshoot, but then a corrective movement overcompensates. The result is an oscillating motion, just as Wiener’s theory would predict when the feedback loop is impaired; Rosenblueth, Wiener, and Bigelow 1943 ↩.
Corcoran and Conner 2016 ↩.
Glimcher 2005; Faisal, Selen, and Wolpert 2008; Braun 2021 ↩, ↩, ↩.
Domenici et al. 2008 ↩.
Genevieve Bell, an anthropologist and Vice-Chancellor of the Australian National University (ANU), founded ANU’s School of Cybernetics in 2021. I wholeheartedly endorse her revival of the term “cybernetics” to include not only AI but also the larger set of multidisciplinary topics touched on here.
Rosenblueth, Wiener, and Bigelow 1943 ↩.
The authors dodge the issue, writing, “A discussion of causality, determinism, and final causes is beyond the scope of this essay […] however […] purposefulness, as defined here, is quite independent of causality, initial or final.” For the moment, we’re also sidestepping the random element inherent to quantum mechanics, though we will return to randomness later.
Philosopher Daniel Dennett has called this, or something much like it, the “intentional stance.” Dennett 1989 ↩.
Rosenblueth, Wiener, and Bigelow 1943 ↩.
MacKenzie 1993 ↩.
Weizenbaum 1966 ↩.
Weizenbaum 1966 ↩.
North 1977 ↩.
Espejo 2014 ↩.
Meadows et al. 1972 ↩.
Jacobs 1961 ↩.
Haraway 1985 ↩.
Clynes and Kline 1960 ↩.
Rid 2016 ↩.
A few years later, in the Soviet Union, cybernetics underwent a parallel rise and fall; see Peters 2016 ↩.
Dreyfus 1972 ↩.
James 1890 ↩. The salient chapter (IV—Habit) was published earlier, in 1887.
Ferreira, Nogueira, and Defelipe 2014 ↩.
Pavlov 1902 ↩.
Pitts and McCulloch 1947 ↩.
Borges 1942 ↩.
Hubel and Wiesel 2004 ↩.
Livingstone 2013 ↩.
Until the late 2010s, video games drove the market for GPUs.
The massively parallel Connection Machine, invented by Danny Hillis and colleagues in the 1980s, was a notable exception, consisting of an array of small, local processors wired up to their neighbors in a 3D torus. As a “connectionist,” Hillis and his colleagues were convinced that their architecture was the future of computing—and specifically of AI. They were too far ahead of the curve, though. Precisely because the Connection Machine was such an architectural outlier, there was never a viable software or research ecosystem for it; see Hillis 1985 ↩.
Schmidhuber 2014 ↩.
Minsky and Papert 1969 ↩.
Cybenko 1989 ↩.
Per Kurzweil 2024 ↩, “[B]ack in 1964 Rosenblatt explained to me that the Perceptron’s inability to deal with invariance was due to a lack of layers. If you took the output of a Perceptron and fed it back to another layer just like it, the output would be more general and, with repeated iterations of this process, would increasingly be able to deal with invariance. If you had enough layers and enough training data, it could deal with an amazing level of complexity. I asked him whether he had actually tried this, and he said no but that it was high on his research agenda. It was an amazing insight, but Rosenblatt died only seven years later, in 1971, before he got the chance to test his insights.”
Sejnowski and Rosenberg 1987 ↩.
By “distributed representations,” the authors meant representations of concepts based on the values of many neurons, also known as neural “embeddings.” Neural embeddings will be described in detail in “Unkneading,” chapter 4.
Minsky and Papert 1988 ↩.
Bender and Koller 2020; Chomsky, Roberts, and Watumull 2023 ↩, ↩.
This, at least, was the longstanding assumption in statistical modeling. The reality turns out to be more complex, as will be described in “Long Tails,” chapter 9; for a modern mathematical treatment of the relationship between data volume and parameter count see Bubeck and Sellke 2022 ↩.
A complete list would be impossible to make, especially without some historical distance; an enormous volume of papers are being published today advocating for one new twist or another. Years can elapse before we know which of these will matter, and sometimes a trick must be reinvented multiple times, with minor variations, before being widely adopted. This process bears more than a passing resemblance to evolution.
LeCun, Denker, and Solla 1989 ↩.
In 2014, “very deep” meant between sixteen and nineteen layers (Simonyan and Zisserman 2014 ↩); by 2015, it meant 152 layers (He et al. 2016a ↩); and in 2016, researchers at Microsoft reported a 1,001-layer network (He et al. 2016b ↩).
Yamaguchi et al. 1990 ↩.
LeCun et al. 1995 ↩.
The annual NeurIPS meeting, which has since grown into a behemoth, was founded in 1987 specifically to focus on both “natural and synthetic” neural information processing systems; Abu-Mostafa 2020 ↩.
Norbert Wiener, Warren McCulloch, Walter Pitts, Frank Rosenblatt, Kunihiko Fukushima, Alan Turing, Geoffrey Hinton, Terry Sejnowski, Rich Sutton, Karl Friston, Yann LeCun, Read Montague, Jürgen Schmidhuber, Sara Solla, Yoshua Bengio, and Peter Dayan are all important figures in the development of machine learning mentioned in this book who work or worked in the tradition of computational neuroscience, or NeuroAI.
Krizhevsky, Sutskever, and Hinton 2012 ↩.
Zhang, McLoughlin, and Song 2015 ↩.
Weyn, Durran, and Caruana 2020 ↩.
Dauphin et al. 2017 ↩.
A flip-flop is a basic electrical circuit that can “flip” into one state, or “flop” into another; thus it is invaluable for implementing digital logic. A multi-stable flip-flop has more than two stable states.
Rosenblatt 1957 ↩.
More technically, any sufficiently continuous function.
Daubechies et al. 2022 ↩.
The term “inductive bias” has been used to describe the latent structural assumptions made by an approximator (whether neural or otherwise) to learn a function from a finite set of examples.
Many refinements to the basic perceptron architecture, including convolutions, different nonlinearities, “skip connections” (He et al. 2016a ↩), and so on can be understood as further biasing the class of functions to be learned, with the goal of making the learning more efficient (that is, requiring fewer parameters or training examples to achieve similar accuracy).
Bojarski et al. 2016 ↩.
This idea had been tried before, with some success. ALVINN, the Autonomous Land Vehicle in a Neural Network (Pomerleau 1989 ↩), was a much smaller, fully connected three-layer perceptron built in 1988—the year Minsky and Papert reissued Perceptrons—and could “effectively follow real roads under certain field conditions,” a milestone GOFAI systems had not (and have not) achieved.
Rosenblatt 1957 ↩.
However, we’ll return to these points when discussing the phenomenon of “in-context learning” in chapter 9.